By: Meenakshi Das, nonprofit analytics consultant
Here is the danger of extreme personalization — we risk limiting ourselves to what we know, what we understand, and what makes us comfortable.
Our complex and imperfect world.
Learning to form a healthy relationship with data is, therefore, a necessity to building equity. Because that establishes our commitment to count right and our patterns of engagement with data. To be counted in that data should not be a matter of power or privilege for anyone.
It must be fundamental.
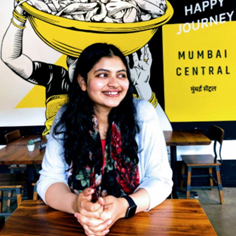
Meenakshi Das
Meenakshi (Meena) Das (she/her/hers) is the founder, data consultant, trainer, and an ethicist with her nonprofit consulting practice, NamasteData. She specializes in designing and teaching equitable research tools and analyzing engagement. Her two workshops referenced in the article are: Advancing Equitable Data Collection and Advancing Equity through Visualizations. Meena appreciates spending her time outside work as a mentor to immigrants. Her recent favorite project is talking about IDEA-led data and research through her LinkedIn-based newsletter data uncollected.
Our complex and imperfect world.
Learning to form a healthy relationship with data is, therefore, a necessity to building equity. Because that establishes our commitment to count right and our patterns of engagement with data. To be counted in that data should not be a matter of power or privilege for anyone.
It must be fundamental.
[/et_pb_text][/et_pb_column][/et_pb_row]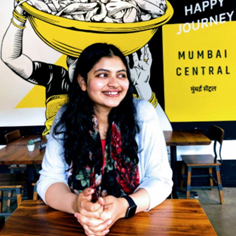
Meenakshi Das
Meenakshi (Meena) Das (she/her/hers) is the founder, data consultant, trainer, and an ethicist with her nonprofit consulting practice, NamasteData. She specializes in designing and teaching equitable research tools and analyzing engagement. Her two workshops referenced in the article are: Advancing Equitable Data Collection and Advancing Equity through Visualizations. Meena appreciates spending her time outside work as a mentor to immigrants. Her recent favorite project is talking about IDEA-led data and research through her LinkedIn-based newsletter data uncollected.
You and I live in a time when data is constantly and continuously collected about us, through us, claiming it is for us. We need to remember that data in itself is not magic. Just like my Netflix recommendation algorithm was dependent on my (previously) limited engagements on the platform, our data outputs will only be as dynamic as the information we give it. It is not perfect, either. It (data) is merely a recorded value — by a person or a device — in this world.
Our complex and imperfect world.
Learning to form a healthy relationship with data is, therefore, a necessity to building equity. Because that establishes our commitment to count right and our patterns of engagement with data. To be counted in that data should not be a matter of power or privilege for anyone.
It must be fundamental.
[/et_pb_text][/et_pb_column][/et_pb_row]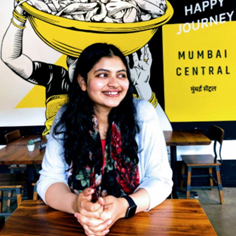
Meenakshi Das
Meenakshi (Meena) Das (she/her/hers) is the founder, data consultant, trainer, and an ethicist with her nonprofit consulting practice, NamasteData. She specializes in designing and teaching equitable research tools and analyzing engagement. Her two workshops referenced in the article are: Advancing Equitable Data Collection and Advancing Equity through Visualizations. Meena appreciates spending her time outside work as a mentor to immigrants. Her recent favorite project is talking about IDEA-led data and research through her LinkedIn-based newsletter data uncollected.
Remember, the “impact” of the stories is not through the numbers but the people in those stories. In the example above, a fair share of voices were included, right from the way data was collected to how it translated into impact. Let us lean on both the data and the people underlying it.
You and I live in a time when data is constantly and continuously collected about us, through us, claiming it is for us. We need to remember that data in itself is not magic. Just like my Netflix recommendation algorithm was dependent on my (previously) limited engagements on the platform, our data outputs will only be as dynamic as the information we give it. It is not perfect, either. It (data) is merely a recorded value — by a person or a device — in this world.
Our complex and imperfect world.
Learning to form a healthy relationship with data is, therefore, a necessity to building equity. Because that establishes our commitment to count right and our patterns of engagement with data. To be counted in that data should not be a matter of power or privilege for anyone.
It must be fundamental.
[/et_pb_text][/et_pb_column][/et_pb_row]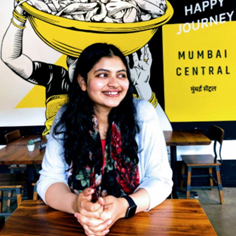
Meenakshi Das
Meenakshi (Meena) Das (she/her/hers) is the founder, data consultant, trainer, and an ethicist with her nonprofit consulting practice, NamasteData. She specializes in designing and teaching equitable research tools and analyzing engagement. Her two workshops referenced in the article are: Advancing Equitable Data Collection and Advancing Equity through Visualizations. Meena appreciates spending her time outside work as a mentor to immigrants. Her recent favorite project is talking about IDEA-led data and research through her LinkedIn-based newsletter data uncollected.
I particularly love a relevant example I received in the workshop. One of the participants was both an annual-giving fundraiser and the data person in the fundraising team of an independent school. Their mission was to offer learning and development opportunities to kids and youth of low-income families with one or both BIPOC parents. She shared how the impact stories they collected in their data didn’t feel adequate in light of the pandemic challenges, so they changed their process. Instead of looking at their own previously collected data, they opened up the process to include their entire community (families, kids, digital supporters, etc.). Not only did they bring representatives of the community in designing the questions of data collection, but they also supported in summarizing and interpreting the responses to define “impact.”
Remember, the “impact” of the stories is not through the numbers but the people in those stories. In the example above, a fair share of voices were included, right from the way data was collected to how it translated into impact. Let us lean on both the data and the people underlying it.
You and I live in a time when data is constantly and continuously collected about us, through us, claiming it is for us. We need to remember that data in itself is not magic. Just like my Netflix recommendation algorithm was dependent on my (previously) limited engagements on the platform, our data outputs will only be as dynamic as the information we give it. It is not perfect, either. It (data) is merely a recorded value — by a person or a device — in this world.
Our complex and imperfect world.
Learning to form a healthy relationship with data is, therefore, a necessity to building equity. Because that establishes our commitment to count right and our patterns of engagement with data. To be counted in that data should not be a matter of power or privilege for anyone.
It must be fundamental.
[/et_pb_text][/et_pb_column][/et_pb_row]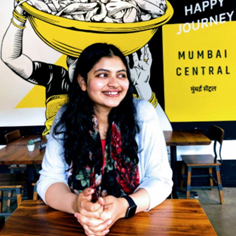
Meenakshi Das
Meenakshi (Meena) Das (she/her/hers) is the founder, data consultant, trainer, and an ethicist with her nonprofit consulting practice, NamasteData. She specializes in designing and teaching equitable research tools and analyzing engagement. Her two workshops referenced in the article are: Advancing Equitable Data Collection and Advancing Equity through Visualizations. Meena appreciates spending her time outside work as a mentor to immigrants. Her recent favorite project is talking about IDEA-led data and research through her LinkedIn-based newsletter data uncollected.
5. All of us who work in the sector are, to a certain extent, storytellers.
We tell stories using the data we have, for the data we want to collect. We can make those stories inclusive by creating a space of collaboration and feedback with the community whose stories they are while learning to say “I don’t know” at appropriate times.
I particularly love a relevant example I received in the workshop. One of the participants was both an annual-giving fundraiser and the data person in the fundraising team of an independent school. Their mission was to offer learning and development opportunities to kids and youth of low-income families with one or both BIPOC parents. She shared how the impact stories they collected in their data didn’t feel adequate in light of the pandemic challenges, so they changed their process. Instead of looking at their own previously collected data, they opened up the process to include their entire community (families, kids, digital supporters, etc.). Not only did they bring representatives of the community in designing the questions of data collection, but they also supported in summarizing and interpreting the responses to define “impact.”
Remember, the “impact” of the stories is not through the numbers but the people in those stories. In the example above, a fair share of voices were included, right from the way data was collected to how it translated into impact. Let us lean on both the data and the people underlying it.
You and I live in a time when data is constantly and continuously collected about us, through us, claiming it is for us. We need to remember that data in itself is not magic. Just like my Netflix recommendation algorithm was dependent on my (previously) limited engagements on the platform, our data outputs will only be as dynamic as the information we give it. It is not perfect, either. It (data) is merely a recorded value — by a person or a device — in this world.
Our complex and imperfect world.
Learning to form a healthy relationship with data is, therefore, a necessity to building equity. Because that establishes our commitment to count right and our patterns of engagement with data. To be counted in that data should not be a matter of power or privilege for anyone.
It must be fundamental.
[/et_pb_text][/et_pb_column][/et_pb_row]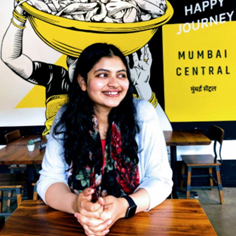
Meenakshi Das
Meenakshi (Meena) Das (she/her/hers) is the founder, data consultant, trainer, and an ethicist with her nonprofit consulting practice, NamasteData. She specializes in designing and teaching equitable research tools and analyzing engagement. Her two workshops referenced in the article are: Advancing Equitable Data Collection and Advancing Equity through Visualizations. Meena appreciates spending her time outside work as a mentor to immigrants. Her recent favorite project is talking about IDEA-led data and research through her LinkedIn-based newsletter data uncollected.
Regardless of how that individual relationship (with data) looks like, the underlying intention here goes back to the first point — reflecting (and acting) on what equity in data means. And, when we make an active effort in understanding “data” and its nuances, we can acknowledge the positional power we have in influencing our data culture.
5. All of us who work in the sector are, to a certain extent, storytellers.
We tell stories using the data we have, for the data we want to collect. We can make those stories inclusive by creating a space of collaboration and feedback with the community whose stories they are while learning to say “I don’t know” at appropriate times.
I particularly love a relevant example I received in the workshop. One of the participants was both an annual-giving fundraiser and the data person in the fundraising team of an independent school. Their mission was to offer learning and development opportunities to kids and youth of low-income families with one or both BIPOC parents. She shared how the impact stories they collected in their data didn’t feel adequate in light of the pandemic challenges, so they changed their process. Instead of looking at their own previously collected data, they opened up the process to include their entire community (families, kids, digital supporters, etc.). Not only did they bring representatives of the community in designing the questions of data collection, but they also supported in summarizing and interpreting the responses to define “impact.”
Remember, the “impact” of the stories is not through the numbers but the people in those stories. In the example above, a fair share of voices were included, right from the way data was collected to how it translated into impact. Let us lean on both the data and the people underlying it.
You and I live in a time when data is constantly and continuously collected about us, through us, claiming it is for us. We need to remember that data in itself is not magic. Just like my Netflix recommendation algorithm was dependent on my (previously) limited engagements on the platform, our data outputs will only be as dynamic as the information we give it. It is not perfect, either. It (data) is merely a recorded value — by a person or a device — in this world.
Our complex and imperfect world.
Learning to form a healthy relationship with data is, therefore, a necessity to building equity. Because that establishes our commitment to count right and our patterns of engagement with data. To be counted in that data should not be a matter of power or privilege for anyone.
It must be fundamental.
[/et_pb_text][/et_pb_column][/et_pb_row]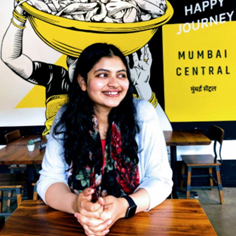
Meenakshi Das
Meenakshi (Meena) Das (she/her/hers) is the founder, data consultant, trainer, and an ethicist with her nonprofit consulting practice, NamasteData. She specializes in designing and teaching equitable research tools and analyzing engagement. Her two workshops referenced in the article are: Advancing Equitable Data Collection and Advancing Equity through Visualizations. Meena appreciates spending her time outside work as a mentor to immigrants. Her recent favorite project is talking about IDEA-led data and research through her LinkedIn-based newsletter data uncollected.
Each of us is responsible for building a better individual relationship with data. This will look different from role to role. A data analyst will require a different level of understanding in cleaning and analyzing data than a team leader responsible to synthesize a bulky report for the Board. Each will require a different lens on the summarized charts and language of its narratives.
Regardless of how that individual relationship (with data) looks like, the underlying intention here goes back to the first point — reflecting (and acting) on what equity in data means. And, when we make an active effort in understanding “data” and its nuances, we can acknowledge the positional power we have in influencing our data culture.
5. All of us who work in the sector are, to a certain extent, storytellers.
We tell stories using the data we have, for the data we want to collect. We can make those stories inclusive by creating a space of collaboration and feedback with the community whose stories they are while learning to say “I don’t know” at appropriate times.
I particularly love a relevant example I received in the workshop. One of the participants was both an annual-giving fundraiser and the data person in the fundraising team of an independent school. Their mission was to offer learning and development opportunities to kids and youth of low-income families with one or both BIPOC parents. She shared how the impact stories they collected in their data didn’t feel adequate in light of the pandemic challenges, so they changed their process. Instead of looking at their own previously collected data, they opened up the process to include their entire community (families, kids, digital supporters, etc.). Not only did they bring representatives of the community in designing the questions of data collection, but they also supported in summarizing and interpreting the responses to define “impact.”
Remember, the “impact” of the stories is not through the numbers but the people in those stories. In the example above, a fair share of voices were included, right from the way data was collected to how it translated into impact. Let us lean on both the data and the people underlying it.
You and I live in a time when data is constantly and continuously collected about us, through us, claiming it is for us. We need to remember that data in itself is not magic. Just like my Netflix recommendation algorithm was dependent on my (previously) limited engagements on the platform, our data outputs will only be as dynamic as the information we give it. It is not perfect, either. It (data) is merely a recorded value — by a person or a device — in this world.
Our complex and imperfect world.
Learning to form a healthy relationship with data is, therefore, a necessity to building equity. Because that establishes our commitment to count right and our patterns of engagement with data. To be counted in that data should not be a matter of power or privilege for anyone.
It must be fundamental.
[/et_pb_text][/et_pb_column][/et_pb_row]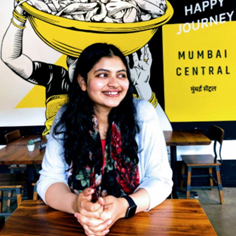
Meenakshi Das
Meenakshi (Meena) Das (she/her/hers) is the founder, data consultant, trainer, and an ethicist with her nonprofit consulting practice, NamasteData. She specializes in designing and teaching equitable research tools and analyzing engagement. Her two workshops referenced in the article are: Advancing Equitable Data Collection and Advancing Equity through Visualizations. Meena appreciates spending her time outside work as a mentor to immigrants. Her recent favorite project is talking about IDEA-led data and research through her LinkedIn-based newsletter data uncollected.
For example, one of the workshop participants once shared how she wanted to update what is collected and reported around the identity of donors, volunteers, and other key groups in her organization. She realized that updates required conversations within and outside her team, so a clear why, what, and how could be collectively established in making those updates. So, she asked for those meetings and space in meeting agendas. But, as an entry-level data coordinator (and a Black woman), she shared how she found resistance to those needed conversations. The updates are still pending and live in an internal tracker.
Each of us is responsible for building a better individual relationship with data. This will look different from role to role. A data analyst will require a different level of understanding in cleaning and analyzing data than a team leader responsible to synthesize a bulky report for the Board. Each will require a different lens on the summarized charts and language of its narratives.
Regardless of how that individual relationship (with data) looks like, the underlying intention here goes back to the first point — reflecting (and acting) on what equity in data means. And, when we make an active effort in understanding “data” and its nuances, we can acknowledge the positional power we have in influencing our data culture.
5. All of us who work in the sector are, to a certain extent, storytellers.
We tell stories using the data we have, for the data we want to collect. We can make those stories inclusive by creating a space of collaboration and feedback with the community whose stories they are while learning to say “I don’t know” at appropriate times.
I particularly love a relevant example I received in the workshop. One of the participants was both an annual-giving fundraiser and the data person in the fundraising team of an independent school. Their mission was to offer learning and development opportunities to kids and youth of low-income families with one or both BIPOC parents. She shared how the impact stories they collected in their data didn’t feel adequate in light of the pandemic challenges, so they changed their process. Instead of looking at their own previously collected data, they opened up the process to include their entire community (families, kids, digital supporters, etc.). Not only did they bring representatives of the community in designing the questions of data collection, but they also supported in summarizing and interpreting the responses to define “impact.”
Remember, the “impact” of the stories is not through the numbers but the people in those stories. In the example above, a fair share of voices were included, right from the way data was collected to how it translated into impact. Let us lean on both the data and the people underlying it.
You and I live in a time when data is constantly and continuously collected about us, through us, claiming it is for us. We need to remember that data in itself is not magic. Just like my Netflix recommendation algorithm was dependent on my (previously) limited engagements on the platform, our data outputs will only be as dynamic as the information we give it. It is not perfect, either. It (data) is merely a recorded value — by a person or a device — in this world.
Our complex and imperfect world.
Learning to form a healthy relationship with data is, therefore, a necessity to building equity. Because that establishes our commitment to count right and our patterns of engagement with data. To be counted in that data should not be a matter of power or privilege for anyone.
It must be fundamental.
[/et_pb_text][/et_pb_column][/et_pb_row]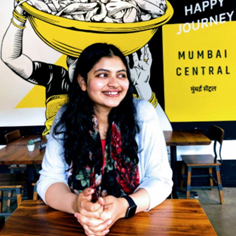
Meenakshi Das
Meenakshi (Meena) Das (she/her/hers) is the founder, data consultant, trainer, and an ethicist with her nonprofit consulting practice, NamasteData. She specializes in designing and teaching equitable research tools and analyzing engagement. Her two workshops referenced in the article are: Advancing Equitable Data Collection and Advancing Equity through Visualizations. Meena appreciates spending her time outside work as a mentor to immigrants. Her recent favorite project is talking about IDEA-led data and research through her LinkedIn-based newsletter data uncollected.
What is the context here, so we understand qualitative vs. quantitative is better?
- Who collected this data?
- Are there actions on the part of the sponsors in ethical use, distribution, and next steps from this data?
- How was this data collected?
- Who will the insights speak to?
When working with data, there is no specific step where equity needs to be centered; equity should be embedded in the entire process. The point of this conversation is to bring awareness and responsibility to our actions for and from our data.
4. Power is deeply intertwined with language.
Changing the data culture to use data responsibly comes with its roadblocks. Several challenges can surface depending on who is leading those efforts in the organization. Some specific roadblocks could be an inability to get before the right stakeholders, being gaslighted by the right stakeholders, or being shut down by someone who holds more power.
For example, one of the workshop participants once shared how she wanted to update what is collected and reported around the identity of donors, volunteers, and other key groups in her organization. She realized that updates required conversations within and outside her team, so a clear why, what, and how could be collectively established in making those updates. So, she asked for those meetings and space in meeting agendas. But, as an entry-level data coordinator (and a Black woman), she shared how she found resistance to those needed conversations. The updates are still pending and live in an internal tracker.
Each of us is responsible for building a better individual relationship with data. This will look different from role to role. A data analyst will require a different level of understanding in cleaning and analyzing data than a team leader responsible to synthesize a bulky report for the Board. Each will require a different lens on the summarized charts and language of its narratives.
Regardless of how that individual relationship (with data) looks like, the underlying intention here goes back to the first point — reflecting (and acting) on what equity in data means. And, when we make an active effort in understanding “data” and its nuances, we can acknowledge the positional power we have in influencing our data culture.
5. All of us who work in the sector are, to a certain extent, storytellers.
We tell stories using the data we have, for the data we want to collect. We can make those stories inclusive by creating a space of collaboration and feedback with the community whose stories they are while learning to say “I don’t know” at appropriate times.
I particularly love a relevant example I received in the workshop. One of the participants was both an annual-giving fundraiser and the data person in the fundraising team of an independent school. Their mission was to offer learning and development opportunities to kids and youth of low-income families with one or both BIPOC parents. She shared how the impact stories they collected in their data didn’t feel adequate in light of the pandemic challenges, so they changed their process. Instead of looking at their own previously collected data, they opened up the process to include their entire community (families, kids, digital supporters, etc.). Not only did they bring representatives of the community in designing the questions of data collection, but they also supported in summarizing and interpreting the responses to define “impact.”
Remember, the “impact” of the stories is not through the numbers but the people in those stories. In the example above, a fair share of voices were included, right from the way data was collected to how it translated into impact. Let us lean on both the data and the people underlying it.
You and I live in a time when data is constantly and continuously collected about us, through us, claiming it is for us. We need to remember that data in itself is not magic. Just like my Netflix recommendation algorithm was dependent on my (previously) limited engagements on the platform, our data outputs will only be as dynamic as the information we give it. It is not perfect, either. It (data) is merely a recorded value — by a person or a device — in this world.
Our complex and imperfect world.
Learning to form a healthy relationship with data is, therefore, a necessity to building equity. Because that establishes our commitment to count right and our patterns of engagement with data. To be counted in that data should not be a matter of power or privilege for anyone.
It must be fundamental.
[/et_pb_text][/et_pb_column][/et_pb_row]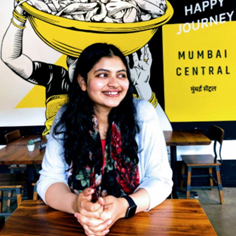
Meenakshi Das
Meenakshi (Meena) Das (she/her/hers) is the founder, data consultant, trainer, and an ethicist with her nonprofit consulting practice, NamasteData. She specializes in designing and teaching equitable research tools and analyzing engagement. Her two workshops referenced in the article are: Advancing Equitable Data Collection and Advancing Equity through Visualizations. Meena appreciates spending her time outside work as a mentor to immigrants. Her recent favorite project is talking about IDEA-led data and research through her LinkedIn-based newsletter data uncollected.
What I bring back from that example into my workshop discussion is – being mindful about the reports that come out of such data collection instances when there are many different voices in the play. When interpreting and translating that data into insights, it is critical to include:
What is the context here, so we understand qualitative vs. quantitative is better?
- Who collected this data?
- Are there actions on the part of the sponsors in ethical use, distribution, and next steps from this data?
- How was this data collected?
- Who will the insights speak to?
When working with data, there is no specific step where equity needs to be centered; equity should be embedded in the entire process. The point of this conversation is to bring awareness and responsibility to our actions for and from our data.
4. Power is deeply intertwined with language.
Changing the data culture to use data responsibly comes with its roadblocks. Several challenges can surface depending on who is leading those efforts in the organization. Some specific roadblocks could be an inability to get before the right stakeholders, being gaslighted by the right stakeholders, or being shut down by someone who holds more power.
For example, one of the workshop participants once shared how she wanted to update what is collected and reported around the identity of donors, volunteers, and other key groups in her organization. She realized that updates required conversations within and outside her team, so a clear why, what, and how could be collectively established in making those updates. So, she asked for those meetings and space in meeting agendas. But, as an entry-level data coordinator (and a Black woman), she shared how she found resistance to those needed conversations. The updates are still pending and live in an internal tracker.
Each of us is responsible for building a better individual relationship with data. This will look different from role to role. A data analyst will require a different level of understanding in cleaning and analyzing data than a team leader responsible to synthesize a bulky report for the Board. Each will require a different lens on the summarized charts and language of its narratives.
Regardless of how that individual relationship (with data) looks like, the underlying intention here goes back to the first point — reflecting (and acting) on what equity in data means. And, when we make an active effort in understanding “data” and its nuances, we can acknowledge the positional power we have in influencing our data culture.
5. All of us who work in the sector are, to a certain extent, storytellers.
We tell stories using the data we have, for the data we want to collect. We can make those stories inclusive by creating a space of collaboration and feedback with the community whose stories they are while learning to say “I don’t know” at appropriate times.
I particularly love a relevant example I received in the workshop. One of the participants was both an annual-giving fundraiser and the data person in the fundraising team of an independent school. Their mission was to offer learning and development opportunities to kids and youth of low-income families with one or both BIPOC parents. She shared how the impact stories they collected in their data didn’t feel adequate in light of the pandemic challenges, so they changed their process. Instead of looking at their own previously collected data, they opened up the process to include their entire community (families, kids, digital supporters, etc.). Not only did they bring representatives of the community in designing the questions of data collection, but they also supported in summarizing and interpreting the responses to define “impact.”
Remember, the “impact” of the stories is not through the numbers but the people in those stories. In the example above, a fair share of voices were included, right from the way data was collected to how it translated into impact. Let us lean on both the data and the people underlying it.
You and I live in a time when data is constantly and continuously collected about us, through us, claiming it is for us. We need to remember that data in itself is not magic. Just like my Netflix recommendation algorithm was dependent on my (previously) limited engagements on the platform, our data outputs will only be as dynamic as the information we give it. It is not perfect, either. It (data) is merely a recorded value — by a person or a device — in this world.
Our complex and imperfect world.
Learning to form a healthy relationship with data is, therefore, a necessity to building equity. Because that establishes our commitment to count right and our patterns of engagement with data. To be counted in that data should not be a matter of power or privilege for anyone.
It must be fundamental.
[/et_pb_text][/et_pb_column][/et_pb_row]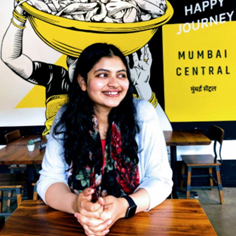
Meenakshi Das
Meenakshi (Meena) Das (she/her/hers) is the founder, data consultant, trainer, and an ethicist with her nonprofit consulting practice, NamasteData. She specializes in designing and teaching equitable research tools and analyzing engagement. Her two workshops referenced in the article are: Advancing Equitable Data Collection and Advancing Equity through Visualizations. Meena appreciates spending her time outside work as a mentor to immigrants. Her recent favorite project is talking about IDEA-led data and research through her LinkedIn-based newsletter data uncollected.
Clearly, the same question generates different reactions (and data points).
What I bring back from that example into my workshop discussion is – being mindful about the reports that come out of such data collection instances when there are many different voices in the play. When interpreting and translating that data into insights, it is critical to include:
What is the context here, so we understand qualitative vs. quantitative is better?
- Who collected this data?
- Are there actions on the part of the sponsors in ethical use, distribution, and next steps from this data?
- How was this data collected?
- Who will the insights speak to?
When working with data, there is no specific step where equity needs to be centered; equity should be embedded in the entire process. The point of this conversation is to bring awareness and responsibility to our actions for and from our data.
4. Power is deeply intertwined with language.
Changing the data culture to use data responsibly comes with its roadblocks. Several challenges can surface depending on who is leading those efforts in the organization. Some specific roadblocks could be an inability to get before the right stakeholders, being gaslighted by the right stakeholders, or being shut down by someone who holds more power.
For example, one of the workshop participants once shared how she wanted to update what is collected and reported around the identity of donors, volunteers, and other key groups in her organization. She realized that updates required conversations within and outside her team, so a clear why, what, and how could be collectively established in making those updates. So, she asked for those meetings and space in meeting agendas. But, as an entry-level data coordinator (and a Black woman), she shared how she found resistance to those needed conversations. The updates are still pending and live in an internal tracker.
Each of us is responsible for building a better individual relationship with data. This will look different from role to role. A data analyst will require a different level of understanding in cleaning and analyzing data than a team leader responsible to synthesize a bulky report for the Board. Each will require a different lens on the summarized charts and language of its narratives.
Regardless of how that individual relationship (with data) looks like, the underlying intention here goes back to the first point — reflecting (and acting) on what equity in data means. And, when we make an active effort in understanding “data” and its nuances, we can acknowledge the positional power we have in influencing our data culture.
5. All of us who work in the sector are, to a certain extent, storytellers.
We tell stories using the data we have, for the data we want to collect. We can make those stories inclusive by creating a space of collaboration and feedback with the community whose stories they are while learning to say “I don’t know” at appropriate times.
I particularly love a relevant example I received in the workshop. One of the participants was both an annual-giving fundraiser and the data person in the fundraising team of an independent school. Their mission was to offer learning and development opportunities to kids and youth of low-income families with one or both BIPOC parents. She shared how the impact stories they collected in their data didn’t feel adequate in light of the pandemic challenges, so they changed their process. Instead of looking at their own previously collected data, they opened up the process to include their entire community (families, kids, digital supporters, etc.). Not only did they bring representatives of the community in designing the questions of data collection, but they also supported in summarizing and interpreting the responses to define “impact.”
Remember, the “impact” of the stories is not through the numbers but the people in those stories. In the example above, a fair share of voices were included, right from the way data was collected to how it translated into impact. Let us lean on both the data and the people underlying it.
You and I live in a time when data is constantly and continuously collected about us, through us, claiming it is for us. We need to remember that data in itself is not magic. Just like my Netflix recommendation algorithm was dependent on my (previously) limited engagements on the platform, our data outputs will only be as dynamic as the information we give it. It is not perfect, either. It (data) is merely a recorded value — by a person or a device — in this world.
Our complex and imperfect world.
Learning to form a healthy relationship with data is, therefore, a necessity to building equity. Because that establishes our commitment to count right and our patterns of engagement with data. To be counted in that data should not be a matter of power or privilege for anyone.
It must be fundamental.
[/et_pb_text][/et_pb_column][/et_pb_row]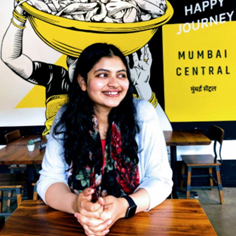
Meenakshi Das
Meenakshi (Meena) Das (she/her/hers) is the founder, data consultant, trainer, and an ethicist with her nonprofit consulting practice, NamasteData. She specializes in designing and teaching equitable research tools and analyzing engagement. Her two workshops referenced in the article are: Advancing Equitable Data Collection and Advancing Equity through Visualizations. Meena appreciates spending her time outside work as a mentor to immigrants. Her recent favorite project is talking about IDEA-led data and research through her LinkedIn-based newsletter data uncollected.
One of the common questions asked in the interviews and focus groups conducted so far has been, “In 2017, a report published 8 out of 10 employers admit ‘regional accents influence recruitment decisions.’ Do you think accents influence job opportunities as first-generation immigrants?” The response is interesting (on the basis of what is collected as of now). There is a clear difference in the response (verbal and body language) when asked to white, native English speakers vs. non-white, multilingual speakers. Similarly, there is a difference in the response when the respondents were first-generation immigrants across the 70s vs 90s vs 2000s, etc.
Clearly, the same question generates different reactions (and data points).
What I bring back from that example into my workshop discussion is – being mindful about the reports that come out of such data collection instances when there are many different voices in the play. When interpreting and translating that data into insights, it is critical to include:
What is the context here, so we understand qualitative vs. quantitative is better?
- Who collected this data?
- Are there actions on the part of the sponsors in ethical use, distribution, and next steps from this data?
- How was this data collected?
- Who will the insights speak to?
When working with data, there is no specific step where equity needs to be centered; equity should be embedded in the entire process. The point of this conversation is to bring awareness and responsibility to our actions for and from our data.
4. Power is deeply intertwined with language.
Changing the data culture to use data responsibly comes with its roadblocks. Several challenges can surface depending on who is leading those efforts in the organization. Some specific roadblocks could be an inability to get before the right stakeholders, being gaslighted by the right stakeholders, or being shut down by someone who holds more power.
For example, one of the workshop participants once shared how she wanted to update what is collected and reported around the identity of donors, volunteers, and other key groups in her organization. She realized that updates required conversations within and outside her team, so a clear why, what, and how could be collectively established in making those updates. So, she asked for those meetings and space in meeting agendas. But, as an entry-level data coordinator (and a Black woman), she shared how she found resistance to those needed conversations. The updates are still pending and live in an internal tracker.
Each of us is responsible for building a better individual relationship with data. This will look different from role to role. A data analyst will require a different level of understanding in cleaning and analyzing data than a team leader responsible to synthesize a bulky report for the Board. Each will require a different lens on the summarized charts and language of its narratives.
Regardless of how that individual relationship (with data) looks like, the underlying intention here goes back to the first point — reflecting (and acting) on what equity in data means. And, when we make an active effort in understanding “data” and its nuances, we can acknowledge the positional power we have in influencing our data culture.
5. All of us who work in the sector are, to a certain extent, storytellers.
We tell stories using the data we have, for the data we want to collect. We can make those stories inclusive by creating a space of collaboration and feedback with the community whose stories they are while learning to say “I don’t know” at appropriate times.
I particularly love a relevant example I received in the workshop. One of the participants was both an annual-giving fundraiser and the data person in the fundraising team of an independent school. Their mission was to offer learning and development opportunities to kids and youth of low-income families with one or both BIPOC parents. She shared how the impact stories they collected in their data didn’t feel adequate in light of the pandemic challenges, so they changed their process. Instead of looking at their own previously collected data, they opened up the process to include their entire community (families, kids, digital supporters, etc.). Not only did they bring representatives of the community in designing the questions of data collection, but they also supported in summarizing and interpreting the responses to define “impact.”
Remember, the “impact” of the stories is not through the numbers but the people in those stories. In the example above, a fair share of voices were included, right from the way data was collected to how it translated into impact. Let us lean on both the data and the people underlying it.
You and I live in a time when data is constantly and continuously collected about us, through us, claiming it is for us. We need to remember that data in itself is not magic. Just like my Netflix recommendation algorithm was dependent on my (previously) limited engagements on the platform, our data outputs will only be as dynamic as the information we give it. It is not perfect, either. It (data) is merely a recorded value — by a person or a device — in this world.
Our complex and imperfect world.
Learning to form a healthy relationship with data is, therefore, a necessity to building equity. Because that establishes our commitment to count right and our patterns of engagement with data. To be counted in that data should not be a matter of power or privilege for anyone.
It must be fundamental.
[/et_pb_text][/et_pb_column][/et_pb_row]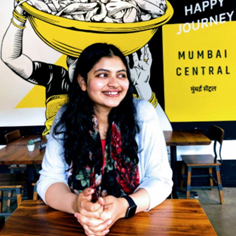
Meenakshi Das
Meenakshi (Meena) Das (she/her/hers) is the founder, data consultant, trainer, and an ethicist with her nonprofit consulting practice, NamasteData. She specializes in designing and teaching equitable research tools and analyzing engagement. Her two workshops referenced in the article are: Advancing Equitable Data Collection and Advancing Equity through Visualizations. Meena appreciates spending her time outside work as a mentor to immigrants. Her recent favorite project is talking about IDEA-led data and research through her LinkedIn-based newsletter data uncollected.
I am working on a research project that involves evaluating if and how the needs of first-generation immigrants across different generations have changed and shaped the local landscape. That project demands both creativity and patience in data collection (it’s an ongoing[MD1] project). It has everything in the mix – interviews, focus groups, and community surveys.
One of the common questions asked in the interviews and focus groups conducted so far has been, “In 2017, a report published 8 out of 10 employers admit ‘regional accents influence recruitment decisions.’ Do you think accents influence job opportunities as first-generation immigrants?” The response is interesting (on the basis of what is collected as of now). There is a clear difference in the response (verbal and body language) when asked to white, native English speakers vs. non-white, multilingual speakers. Similarly, there is a difference in the response when the respondents were first-generation immigrants across the 70s vs 90s vs 2000s, etc.
Clearly, the same question generates different reactions (and data points).
What I bring back from that example into my workshop discussion is – being mindful about the reports that come out of such data collection instances when there are many different voices in the play. When interpreting and translating that data into insights, it is critical to include:
What is the context here, so we understand qualitative vs. quantitative is better?
- Who collected this data?
- Are there actions on the part of the sponsors in ethical use, distribution, and next steps from this data?
- How was this data collected?
- Who will the insights speak to?
When working with data, there is no specific step where equity needs to be centered; equity should be embedded in the entire process. The point of this conversation is to bring awareness and responsibility to our actions for and from our data.
4. Power is deeply intertwined with language.
Changing the data culture to use data responsibly comes with its roadblocks. Several challenges can surface depending on who is leading those efforts in the organization. Some specific roadblocks could be an inability to get before the right stakeholders, being gaslighted by the right stakeholders, or being shut down by someone who holds more power.
For example, one of the workshop participants once shared how she wanted to update what is collected and reported around the identity of donors, volunteers, and other key groups in her organization. She realized that updates required conversations within and outside her team, so a clear why, what, and how could be collectively established in making those updates. So, she asked for those meetings and space in meeting agendas. But, as an entry-level data coordinator (and a Black woman), she shared how she found resistance to those needed conversations. The updates are still pending and live in an internal tracker.
Each of us is responsible for building a better individual relationship with data. This will look different from role to role. A data analyst will require a different level of understanding in cleaning and analyzing data than a team leader responsible to synthesize a bulky report for the Board. Each will require a different lens on the summarized charts and language of its narratives.
Regardless of how that individual relationship (with data) looks like, the underlying intention here goes back to the first point — reflecting (and acting) on what equity in data means. And, when we make an active effort in understanding “data” and its nuances, we can acknowledge the positional power we have in influencing our data culture.
5. All of us who work in the sector are, to a certain extent, storytellers.
We tell stories using the data we have, for the data we want to collect. We can make those stories inclusive by creating a space of collaboration and feedback with the community whose stories they are while learning to say “I don’t know” at appropriate times.
I particularly love a relevant example I received in the workshop. One of the participants was both an annual-giving fundraiser and the data person in the fundraising team of an independent school. Their mission was to offer learning and development opportunities to kids and youth of low-income families with one or both BIPOC parents. She shared how the impact stories they collected in their data didn’t feel adequate in light of the pandemic challenges, so they changed their process. Instead of looking at their own previously collected data, they opened up the process to include their entire community (families, kids, digital supporters, etc.). Not only did they bring representatives of the community in designing the questions of data collection, but they also supported in summarizing and interpreting the responses to define “impact.”
Remember, the “impact” of the stories is not through the numbers but the people in those stories. In the example above, a fair share of voices were included, right from the way data was collected to how it translated into impact. Let us lean on both the data and the people underlying it.
You and I live in a time when data is constantly and continuously collected about us, through us, claiming it is for us. We need to remember that data in itself is not magic. Just like my Netflix recommendation algorithm was dependent on my (previously) limited engagements on the platform, our data outputs will only be as dynamic as the information we give it. It is not perfect, either. It (data) is merely a recorded value — by a person or a device — in this world.
Our complex and imperfect world.
Learning to form a healthy relationship with data is, therefore, a necessity to building equity. Because that establishes our commitment to count right and our patterns of engagement with data. To be counted in that data should not be a matter of power or privilege for anyone.
It must be fundamental.
[/et_pb_text][/et_pb_column][/et_pb_row]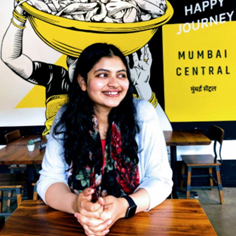
Meenakshi Das
Meenakshi (Meena) Das (she/her/hers) is the founder, data consultant, trainer, and an ethicist with her nonprofit consulting practice, NamasteData. She specializes in designing and teaching equitable research tools and analyzing engagement. Her two workshops referenced in the article are: Advancing Equitable Data Collection and Advancing Equity through Visualizations. Meena appreciates spending her time outside work as a mentor to immigrants. Her recent favorite project is talking about IDEA-led data and research through her LinkedIn-based newsletter data uncollected.
3. The same data may tell very different stories to each group of people it impacts.
Many years ago, my statistics professor taught us a trick. “Add a quantitative data point and you will generate a reaction.” Years later, I got to use it in my project (and now in this example). Here is an anecdote that I often use and discuss in my workshop:
I am working on a research project that involves evaluating if and how the needs of first-generation immigrants across different generations have changed and shaped the local landscape. That project demands both creativity and patience in data collection (it’s an ongoing[MD1] project). It has everything in the mix – interviews, focus groups, and community surveys.
One of the common questions asked in the interviews and focus groups conducted so far has been, “In 2017, a report published 8 out of 10 employers admit ‘regional accents influence recruitment decisions.’ Do you think accents influence job opportunities as first-generation immigrants?” The response is interesting (on the basis of what is collected as of now). There is a clear difference in the response (verbal and body language) when asked to white, native English speakers vs. non-white, multilingual speakers. Similarly, there is a difference in the response when the respondents were first-generation immigrants across the 70s vs 90s vs 2000s, etc.
Clearly, the same question generates different reactions (and data points).
What I bring back from that example into my workshop discussion is – being mindful about the reports that come out of such data collection instances when there are many different voices in the play. When interpreting and translating that data into insights, it is critical to include:
What is the context here, so we understand qualitative vs. quantitative is better?
- Who collected this data?
- Are there actions on the part of the sponsors in ethical use, distribution, and next steps from this data?
- How was this data collected?
- Who will the insights speak to?
When working with data, there is no specific step where equity needs to be centered; equity should be embedded in the entire process. The point of this conversation is to bring awareness and responsibility to our actions for and from our data.
4. Power is deeply intertwined with language.
Changing the data culture to use data responsibly comes with its roadblocks. Several challenges can surface depending on who is leading those efforts in the organization. Some specific roadblocks could be an inability to get before the right stakeholders, being gaslighted by the right stakeholders, or being shut down by someone who holds more power.
For example, one of the workshop participants once shared how she wanted to update what is collected and reported around the identity of donors, volunteers, and other key groups in her organization. She realized that updates required conversations within and outside her team, so a clear why, what, and how could be collectively established in making those updates. So, she asked for those meetings and space in meeting agendas. But, as an entry-level data coordinator (and a Black woman), she shared how she found resistance to those needed conversations. The updates are still pending and live in an internal tracker.
Each of us is responsible for building a better individual relationship with data. This will look different from role to role. A data analyst will require a different level of understanding in cleaning and analyzing data than a team leader responsible to synthesize a bulky report for the Board. Each will require a different lens on the summarized charts and language of its narratives.
Regardless of how that individual relationship (with data) looks like, the underlying intention here goes back to the first point — reflecting (and acting) on what equity in data means. And, when we make an active effort in understanding “data” and its nuances, we can acknowledge the positional power we have in influencing our data culture.
5. All of us who work in the sector are, to a certain extent, storytellers.
We tell stories using the data we have, for the data we want to collect. We can make those stories inclusive by creating a space of collaboration and feedback with the community whose stories they are while learning to say “I don’t know” at appropriate times.
I particularly love a relevant example I received in the workshop. One of the participants was both an annual-giving fundraiser and the data person in the fundraising team of an independent school. Their mission was to offer learning and development opportunities to kids and youth of low-income families with one or both BIPOC parents. She shared how the impact stories they collected in their data didn’t feel adequate in light of the pandemic challenges, so they changed their process. Instead of looking at their own previously collected data, they opened up the process to include their entire community (families, kids, digital supporters, etc.). Not only did they bring representatives of the community in designing the questions of data collection, but they also supported in summarizing and interpreting the responses to define “impact.”
Remember, the “impact” of the stories is not through the numbers but the people in those stories. In the example above, a fair share of voices were included, right from the way data was collected to how it translated into impact. Let us lean on both the data and the people underlying it.
You and I live in a time when data is constantly and continuously collected about us, through us, claiming it is for us. We need to remember that data in itself is not magic. Just like my Netflix recommendation algorithm was dependent on my (previously) limited engagements on the platform, our data outputs will only be as dynamic as the information we give it. It is not perfect, either. It (data) is merely a recorded value — by a person or a device — in this world.
Our complex and imperfect world.
Learning to form a healthy relationship with data is, therefore, a necessity to building equity. Because that establishes our commitment to count right and our patterns of engagement with data. To be counted in that data should not be a matter of power or privilege for anyone.
It must be fundamental.
[/et_pb_text][/et_pb_column][/et_pb_row]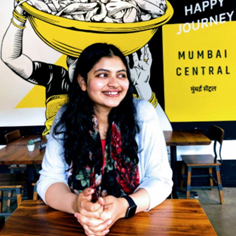
Meenakshi Das
Meenakshi (Meena) Das (she/her/hers) is the founder, data consultant, trainer, and an ethicist with her nonprofit consulting practice, NamasteData. She specializes in designing and teaching equitable research tools and analyzing engagement. Her two workshops referenced in the article are: Advancing Equitable Data Collection and Advancing Equity through Visualizations. Meena appreciates spending her time outside work as a mentor to immigrants. Her recent favorite project is talking about IDEA-led data and research through her LinkedIn-based newsletter data uncollected.
There is missing and broken trust in how we have collected and counted data in the past. And, designing those questions, like always, does not generate confidence. After all, it is something for which people continue to be excluded and harmed. Designing data collection questions around identity information thus starts with two fundamentals. One, as the data collection team, being clear about the purpose of the data that is collected. That purpose has to connect with a concrete action. Example: pronouns are asked so they can be used to appropriately acknowledge individuals in written/verbal communication. Two, as designers, being flexible in the way questions are asked so it prioritizes the people who respond. Example: checkbox vs. open-ended identity-based questions. I acknowledge that this second point (being flexible in design) can be tricky at times, and it is something I get to brainstorm with my co-learners.
3. The same data may tell very different stories to each group of people it impacts.
Many years ago, my statistics professor taught us a trick. “Add a quantitative data point and you will generate a reaction.” Years later, I got to use it in my project (and now in this example). Here is an anecdote that I often use and discuss in my workshop:
I am working on a research project that involves evaluating if and how the needs of first-generation immigrants across different generations have changed and shaped the local landscape. That project demands both creativity and patience in data collection (it’s an ongoing[MD1] project). It has everything in the mix – interviews, focus groups, and community surveys.
One of the common questions asked in the interviews and focus groups conducted so far has been, “In 2017, a report published 8 out of 10 employers admit ‘regional accents influence recruitment decisions.’ Do you think accents influence job opportunities as first-generation immigrants?” The response is interesting (on the basis of what is collected as of now). There is a clear difference in the response (verbal and body language) when asked to white, native English speakers vs. non-white, multilingual speakers. Similarly, there is a difference in the response when the respondents were first-generation immigrants across the 70s vs 90s vs 2000s, etc.
Clearly, the same question generates different reactions (and data points).
What I bring back from that example into my workshop discussion is – being mindful about the reports that come out of such data collection instances when there are many different voices in the play. When interpreting and translating that data into insights, it is critical to include:
What is the context here, so we understand qualitative vs. quantitative is better?
- Who collected this data?
- Are there actions on the part of the sponsors in ethical use, distribution, and next steps from this data?
- How was this data collected?
- Who will the insights speak to?
When working with data, there is no specific step where equity needs to be centered; equity should be embedded in the entire process. The point of this conversation is to bring awareness and responsibility to our actions for and from our data.
4. Power is deeply intertwined with language.
Changing the data culture to use data responsibly comes with its roadblocks. Several challenges can surface depending on who is leading those efforts in the organization. Some specific roadblocks could be an inability to get before the right stakeholders, being gaslighted by the right stakeholders, or being shut down by someone who holds more power.
For example, one of the workshop participants once shared how she wanted to update what is collected and reported around the identity of donors, volunteers, and other key groups in her organization. She realized that updates required conversations within and outside her team, so a clear why, what, and how could be collectively established in making those updates. So, she asked for those meetings and space in meeting agendas. But, as an entry-level data coordinator (and a Black woman), she shared how she found resistance to those needed conversations. The updates are still pending and live in an internal tracker.
Each of us is responsible for building a better individual relationship with data. This will look different from role to role. A data analyst will require a different level of understanding in cleaning and analyzing data than a team leader responsible to synthesize a bulky report for the Board. Each will require a different lens on the summarized charts and language of its narratives.
Regardless of how that individual relationship (with data) looks like, the underlying intention here goes back to the first point — reflecting (and acting) on what equity in data means. And, when we make an active effort in understanding “data” and its nuances, we can acknowledge the positional power we have in influencing our data culture.
5. All of us who work in the sector are, to a certain extent, storytellers.
We tell stories using the data we have, for the data we want to collect. We can make those stories inclusive by creating a space of collaboration and feedback with the community whose stories they are while learning to say “I don’t know” at appropriate times.
I particularly love a relevant example I received in the workshop. One of the participants was both an annual-giving fundraiser and the data person in the fundraising team of an independent school. Their mission was to offer learning and development opportunities to kids and youth of low-income families with one or both BIPOC parents. She shared how the impact stories they collected in their data didn’t feel adequate in light of the pandemic challenges, so they changed their process. Instead of looking at their own previously collected data, they opened up the process to include their entire community (families, kids, digital supporters, etc.). Not only did they bring representatives of the community in designing the questions of data collection, but they also supported in summarizing and interpreting the responses to define “impact.”
Remember, the “impact” of the stories is not through the numbers but the people in those stories. In the example above, a fair share of voices were included, right from the way data was collected to how it translated into impact. Let us lean on both the data and the people underlying it.
You and I live in a time when data is constantly and continuously collected about us, through us, claiming it is for us. We need to remember that data in itself is not magic. Just like my Netflix recommendation algorithm was dependent on my (previously) limited engagements on the platform, our data outputs will only be as dynamic as the information we give it. It is not perfect, either. It (data) is merely a recorded value — by a person or a device — in this world.
Our complex and imperfect world.
Learning to form a healthy relationship with data is, therefore, a necessity to building equity. Because that establishes our commitment to count right and our patterns of engagement with data. To be counted in that data should not be a matter of power or privilege for anyone.
It must be fundamental.
[/et_pb_text][/et_pb_column][/et_pb_row]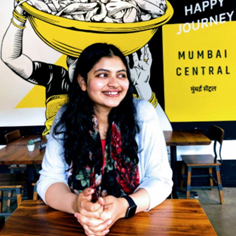
Meenakshi Das
Meenakshi (Meena) Das (she/her/hers) is the founder, data consultant, trainer, and an ethicist with her nonprofit consulting practice, NamasteData. She specializes in designing and teaching equitable research tools and analyzing engagement. Her two workshops referenced in the article are: Advancing Equitable Data Collection and Advancing Equity through Visualizations. Meena appreciates spending her time outside work as a mentor to immigrants. Her recent favorite project is talking about IDEA-led data and research through her LinkedIn-based newsletter data uncollected.
For example, the questions around gender, race, and sexual orientation are often offered in limited 5-7 checkbox options. Identity is extremely personal information about someone, and 5-7 options may not cover everyone’s personal identity.
There is missing and broken trust in how we have collected and counted data in the past. And, designing those questions, like always, does not generate confidence. After all, it is something for which people continue to be excluded and harmed. Designing data collection questions around identity information thus starts with two fundamentals. One, as the data collection team, being clear about the purpose of the data that is collected. That purpose has to connect with a concrete action. Example: pronouns are asked so they can be used to appropriately acknowledge individuals in written/verbal communication. Two, as designers, being flexible in the way questions are asked so it prioritizes the people who respond. Example: checkbox vs. open-ended identity-based questions. I acknowledge that this second point (being flexible in design) can be tricky at times, and it is something I get to brainstorm with my co-learners.
3. The same data may tell very different stories to each group of people it impacts.
Many years ago, my statistics professor taught us a trick. “Add a quantitative data point and you will generate a reaction.” Years later, I got to use it in my project (and now in this example). Here is an anecdote that I often use and discuss in my workshop:
I am working on a research project that involves evaluating if and how the needs of first-generation immigrants across different generations have changed and shaped the local landscape. That project demands both creativity and patience in data collection (it’s an ongoing[MD1] project). It has everything in the mix – interviews, focus groups, and community surveys.
One of the common questions asked in the interviews and focus groups conducted so far has been, “In 2017, a report published 8 out of 10 employers admit ‘regional accents influence recruitment decisions.’ Do you think accents influence job opportunities as first-generation immigrants?” The response is interesting (on the basis of what is collected as of now). There is a clear difference in the response (verbal and body language) when asked to white, native English speakers vs. non-white, multilingual speakers. Similarly, there is a difference in the response when the respondents were first-generation immigrants across the 70s vs 90s vs 2000s, etc.
Clearly, the same question generates different reactions (and data points).
What I bring back from that example into my workshop discussion is – being mindful about the reports that come out of such data collection instances when there are many different voices in the play. When interpreting and translating that data into insights, it is critical to include:
What is the context here, so we understand qualitative vs. quantitative is better?
- Who collected this data?
- Are there actions on the part of the sponsors in ethical use, distribution, and next steps from this data?
- How was this data collected?
- Who will the insights speak to?
When working with data, there is no specific step where equity needs to be centered; equity should be embedded in the entire process. The point of this conversation is to bring awareness and responsibility to our actions for and from our data.
4. Power is deeply intertwined with language.
Changing the data culture to use data responsibly comes with its roadblocks. Several challenges can surface depending on who is leading those efforts in the organization. Some specific roadblocks could be an inability to get before the right stakeholders, being gaslighted by the right stakeholders, or being shut down by someone who holds more power.
For example, one of the workshop participants once shared how she wanted to update what is collected and reported around the identity of donors, volunteers, and other key groups in her organization. She realized that updates required conversations within and outside her team, so a clear why, what, and how could be collectively established in making those updates. So, she asked for those meetings and space in meeting agendas. But, as an entry-level data coordinator (and a Black woman), she shared how she found resistance to those needed conversations. The updates are still pending and live in an internal tracker.
Each of us is responsible for building a better individual relationship with data. This will look different from role to role. A data analyst will require a different level of understanding in cleaning and analyzing data than a team leader responsible to synthesize a bulky report for the Board. Each will require a different lens on the summarized charts and language of its narratives.
Regardless of how that individual relationship (with data) looks like, the underlying intention here goes back to the first point — reflecting (and acting) on what equity in data means. And, when we make an active effort in understanding “data” and its nuances, we can acknowledge the positional power we have in influencing our data culture.
5. All of us who work in the sector are, to a certain extent, storytellers.
We tell stories using the data we have, for the data we want to collect. We can make those stories inclusive by creating a space of collaboration and feedback with the community whose stories they are while learning to say “I don’t know” at appropriate times.
I particularly love a relevant example I received in the workshop. One of the participants was both an annual-giving fundraiser and the data person in the fundraising team of an independent school. Their mission was to offer learning and development opportunities to kids and youth of low-income families with one or both BIPOC parents. She shared how the impact stories they collected in their data didn’t feel adequate in light of the pandemic challenges, so they changed their process. Instead of looking at their own previously collected data, they opened up the process to include their entire community (families, kids, digital supporters, etc.). Not only did they bring representatives of the community in designing the questions of data collection, but they also supported in summarizing and interpreting the responses to define “impact.”
Remember, the “impact” of the stories is not through the numbers but the people in those stories. In the example above, a fair share of voices were included, right from the way data was collected to how it translated into impact. Let us lean on both the data and the people underlying it.
You and I live in a time when data is constantly and continuously collected about us, through us, claiming it is for us. We need to remember that data in itself is not magic. Just like my Netflix recommendation algorithm was dependent on my (previously) limited engagements on the platform, our data outputs will only be as dynamic as the information we give it. It is not perfect, either. It (data) is merely a recorded value — by a person or a device — in this world.
Our complex and imperfect world.
Learning to form a healthy relationship with data is, therefore, a necessity to building equity. Because that establishes our commitment to count right and our patterns of engagement with data. To be counted in that data should not be a matter of power or privilege for anyone.
It must be fundamental.
[/et_pb_text][/et_pb_column][/et_pb_row]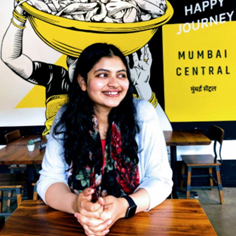
Meenakshi Das
Meenakshi (Meena) Das (she/her/hers) is the founder, data consultant, trainer, and an ethicist with her nonprofit consulting practice, NamasteData. She specializes in designing and teaching equitable research tools and analyzing engagement. Her two workshops referenced in the article are: Advancing Equitable Data Collection and Advancing Equity through Visualizations. Meena appreciates spending her time outside work as a mentor to immigrants. Her recent favorite project is talking about IDEA-led data and research through her LinkedIn-based newsletter data uncollected.
2. Identity is very personal (and fluid at times) information. There is no one right way to capture it, except that it has to be human.
Acknowledge that this is personal and fluid information (fluid because identity information can change). That means it should be a non-negotiable priority to design the questions in a way that centers the people responding to those questions in your data collection exercise.
For example, the questions around gender, race, and sexual orientation are often offered in limited 5-7 checkbox options. Identity is extremely personal information about someone, and 5-7 options may not cover everyone’s personal identity.
There is missing and broken trust in how we have collected and counted data in the past. And, designing those questions, like always, does not generate confidence. After all, it is something for which people continue to be excluded and harmed. Designing data collection questions around identity information thus starts with two fundamentals. One, as the data collection team, being clear about the purpose of the data that is collected. That purpose has to connect with a concrete action. Example: pronouns are asked so they can be used to appropriately acknowledge individuals in written/verbal communication. Two, as designers, being flexible in the way questions are asked so it prioritizes the people who respond. Example: checkbox vs. open-ended identity-based questions. I acknowledge that this second point (being flexible in design) can be tricky at times, and it is something I get to brainstorm with my co-learners.
3. The same data may tell very different stories to each group of people it impacts.
Many years ago, my statistics professor taught us a trick. “Add a quantitative data point and you will generate a reaction.” Years later, I got to use it in my project (and now in this example). Here is an anecdote that I often use and discuss in my workshop:
I am working on a research project that involves evaluating if and how the needs of first-generation immigrants across different generations have changed and shaped the local landscape. That project demands both creativity and patience in data collection (it’s an ongoing[MD1] project). It has everything in the mix – interviews, focus groups, and community surveys.
One of the common questions asked in the interviews and focus groups conducted so far has been, “In 2017, a report published 8 out of 10 employers admit ‘regional accents influence recruitment decisions.’ Do you think accents influence job opportunities as first-generation immigrants?” The response is interesting (on the basis of what is collected as of now). There is a clear difference in the response (verbal and body language) when asked to white, native English speakers vs. non-white, multilingual speakers. Similarly, there is a difference in the response when the respondents were first-generation immigrants across the 70s vs 90s vs 2000s, etc.
Clearly, the same question generates different reactions (and data points).
What I bring back from that example into my workshop discussion is – being mindful about the reports that come out of such data collection instances when there are many different voices in the play. When interpreting and translating that data into insights, it is critical to include:
What is the context here, so we understand qualitative vs. quantitative is better?
- Who collected this data?
- Are there actions on the part of the sponsors in ethical use, distribution, and next steps from this data?
- How was this data collected?
- Who will the insights speak to?
When working with data, there is no specific step where equity needs to be centered; equity should be embedded in the entire process. The point of this conversation is to bring awareness and responsibility to our actions for and from our data.
4. Power is deeply intertwined with language.
Changing the data culture to use data responsibly comes with its roadblocks. Several challenges can surface depending on who is leading those efforts in the organization. Some specific roadblocks could be an inability to get before the right stakeholders, being gaslighted by the right stakeholders, or being shut down by someone who holds more power.
For example, one of the workshop participants once shared how she wanted to update what is collected and reported around the identity of donors, volunteers, and other key groups in her organization. She realized that updates required conversations within and outside her team, so a clear why, what, and how could be collectively established in making those updates. So, she asked for those meetings and space in meeting agendas. But, as an entry-level data coordinator (and a Black woman), she shared how she found resistance to those needed conversations. The updates are still pending and live in an internal tracker.
Each of us is responsible for building a better individual relationship with data. This will look different from role to role. A data analyst will require a different level of understanding in cleaning and analyzing data than a team leader responsible to synthesize a bulky report for the Board. Each will require a different lens on the summarized charts and language of its narratives.
Regardless of how that individual relationship (with data) looks like, the underlying intention here goes back to the first point — reflecting (and acting) on what equity in data means. And, when we make an active effort in understanding “data” and its nuances, we can acknowledge the positional power we have in influencing our data culture.
5. All of us who work in the sector are, to a certain extent, storytellers.
We tell stories using the data we have, for the data we want to collect. We can make those stories inclusive by creating a space of collaboration and feedback with the community whose stories they are while learning to say “I don’t know” at appropriate times.
I particularly love a relevant example I received in the workshop. One of the participants was both an annual-giving fundraiser and the data person in the fundraising team of an independent school. Their mission was to offer learning and development opportunities to kids and youth of low-income families with one or both BIPOC parents. She shared how the impact stories they collected in their data didn’t feel adequate in light of the pandemic challenges, so they changed their process. Instead of looking at their own previously collected data, they opened up the process to include their entire community (families, kids, digital supporters, etc.). Not only did they bring representatives of the community in designing the questions of data collection, but they also supported in summarizing and interpreting the responses to define “impact.”
Remember, the “impact” of the stories is not through the numbers but the people in those stories. In the example above, a fair share of voices were included, right from the way data was collected to how it translated into impact. Let us lean on both the data and the people underlying it.
You and I live in a time when data is constantly and continuously collected about us, through us, claiming it is for us. We need to remember that data in itself is not magic. Just like my Netflix recommendation algorithm was dependent on my (previously) limited engagements on the platform, our data outputs will only be as dynamic as the information we give it. It is not perfect, either. It (data) is merely a recorded value — by a person or a device — in this world.
Our complex and imperfect world.
Learning to form a healthy relationship with data is, therefore, a necessity to building equity. Because that establishes our commitment to count right and our patterns of engagement with data. To be counted in that data should not be a matter of power or privilege for anyone.
It must be fundamental.
[/et_pb_text][/et_pb_column][/et_pb_row]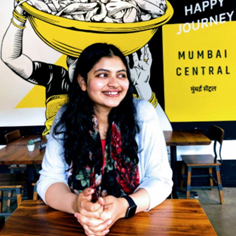
Meenakshi Das
Meenakshi (Meena) Das (she/her/hers) is the founder, data consultant, trainer, and an ethicist with her nonprofit consulting practice, NamasteData. She specializes in designing and teaching equitable research tools and analyzing engagement. Her two workshops referenced in the article are: Advancing Equitable Data Collection and Advancing Equity through Visualizations. Meena appreciates spending her time outside work as a mentor to immigrants. Her recent favorite project is talking about IDEA-led data and research through her LinkedIn-based newsletter data uncollected.
- I want my co-learners to actively reflect on their lived and learned experiences, so they realize how their (and their community’s collective) privileges and challenges are counted or eliminated from the data, and
- I want each of us to remember and be able to distinguish between “IDEA data” and “IDEA-led data.”“IDEA data” is a clear term — it is the data about inclusion, diversity, equity, and accessibility. “IDEA-led data,” on the contrary, is an evolving term — it is about the usage of data in a way that is grounded in the intentions of inclusion, diversity, equity, and accessibility. This usage of data can be in any shape —collection, analysis, strategy development, etc. “IDEA data” can be seen as a subset of “IDEA-led data.”
I must admit that I deeply appreciate the responses we generate as a group in this process. Each response is unique, and yet, all of them together gives “equity” in data a meaning.
See it this way, ask every household member to clean the messiest room and observe how they do it. I can guarantee they won’t have the same starting point in cleaning that room. Some will start with folding laundry; others might start with picking out all empty bottles, cans, or products in the room. That’s what it is like to learn advancing equity in data. That journey is unique and personal — and it starts with learning about diversity, access, and voice. There is no one checklist of “how-to” here. It starts with asking ourselves — what is our purpose and intention with the data? Does it perpetuate harm or create opportunities of equity and inclusion?
2. Identity is very personal (and fluid at times) information. There is no one right way to capture it, except that it has to be human.
Acknowledge that this is personal and fluid information (fluid because identity information can change). That means it should be a non-negotiable priority to design the questions in a way that centers the people responding to those questions in your data collection exercise.
For example, the questions around gender, race, and sexual orientation are often offered in limited 5-7 checkbox options. Identity is extremely personal information about someone, and 5-7 options may not cover everyone’s personal identity.
There is missing and broken trust in how we have collected and counted data in the past. And, designing those questions, like always, does not generate confidence. After all, it is something for which people continue to be excluded and harmed. Designing data collection questions around identity information thus starts with two fundamentals. One, as the data collection team, being clear about the purpose of the data that is collected. That purpose has to connect with a concrete action. Example: pronouns are asked so they can be used to appropriately acknowledge individuals in written/verbal communication. Two, as designers, being flexible in the way questions are asked so it prioritizes the people who respond. Example: checkbox vs. open-ended identity-based questions. I acknowledge that this second point (being flexible in design) can be tricky at times, and it is something I get to brainstorm with my co-learners.
3. The same data may tell very different stories to each group of people it impacts.
Many years ago, my statistics professor taught us a trick. “Add a quantitative data point and you will generate a reaction.” Years later, I got to use it in my project (and now in this example). Here is an anecdote that I often use and discuss in my workshop:
I am working on a research project that involves evaluating if and how the needs of first-generation immigrants across different generations have changed and shaped the local landscape. That project demands both creativity and patience in data collection (it’s an ongoing[MD1] project). It has everything in the mix – interviews, focus groups, and community surveys.
One of the common questions asked in the interviews and focus groups conducted so far has been, “In 2017, a report published 8 out of 10 employers admit ‘regional accents influence recruitment decisions.’ Do you think accents influence job opportunities as first-generation immigrants?” The response is interesting (on the basis of what is collected as of now). There is a clear difference in the response (verbal and body language) when asked to white, native English speakers vs. non-white, multilingual speakers. Similarly, there is a difference in the response when the respondents were first-generation immigrants across the 70s vs 90s vs 2000s, etc.
Clearly, the same question generates different reactions (and data points).
What I bring back from that example into my workshop discussion is – being mindful about the reports that come out of such data collection instances when there are many different voices in the play. When interpreting and translating that data into insights, it is critical to include:
What is the context here, so we understand qualitative vs. quantitative is better?
- Who collected this data?
- Are there actions on the part of the sponsors in ethical use, distribution, and next steps from this data?
- How was this data collected?
- Who will the insights speak to?
When working with data, there is no specific step where equity needs to be centered; equity should be embedded in the entire process. The point of this conversation is to bring awareness and responsibility to our actions for and from our data.
4. Power is deeply intertwined with language.
Changing the data culture to use data responsibly comes with its roadblocks. Several challenges can surface depending on who is leading those efforts in the organization. Some specific roadblocks could be an inability to get before the right stakeholders, being gaslighted by the right stakeholders, or being shut down by someone who holds more power.
For example, one of the workshop participants once shared how she wanted to update what is collected and reported around the identity of donors, volunteers, and other key groups in her organization. She realized that updates required conversations within and outside her team, so a clear why, what, and how could be collectively established in making those updates. So, she asked for those meetings and space in meeting agendas. But, as an entry-level data coordinator (and a Black woman), she shared how she found resistance to those needed conversations. The updates are still pending and live in an internal tracker.
Each of us is responsible for building a better individual relationship with data. This will look different from role to role. A data analyst will require a different level of understanding in cleaning and analyzing data than a team leader responsible to synthesize a bulky report for the Board. Each will require a different lens on the summarized charts and language of its narratives.
Regardless of how that individual relationship (with data) looks like, the underlying intention here goes back to the first point — reflecting (and acting) on what equity in data means. And, when we make an active effort in understanding “data” and its nuances, we can acknowledge the positional power we have in influencing our data culture.
5. All of us who work in the sector are, to a certain extent, storytellers.
We tell stories using the data we have, for the data we want to collect. We can make those stories inclusive by creating a space of collaboration and feedback with the community whose stories they are while learning to say “I don’t know” at appropriate times.
I particularly love a relevant example I received in the workshop. One of the participants was both an annual-giving fundraiser and the data person in the fundraising team of an independent school. Their mission was to offer learning and development opportunities to kids and youth of low-income families with one or both BIPOC parents. She shared how the impact stories they collected in their data didn’t feel adequate in light of the pandemic challenges, so they changed their process. Instead of looking at their own previously collected data, they opened up the process to include their entire community (families, kids, digital supporters, etc.). Not only did they bring representatives of the community in designing the questions of data collection, but they also supported in summarizing and interpreting the responses to define “impact.”
Remember, the “impact” of the stories is not through the numbers but the people in those stories. In the example above, a fair share of voices were included, right from the way data was collected to how it translated into impact. Let us lean on both the data and the people underlying it.
You and I live in a time when data is constantly and continuously collected about us, through us, claiming it is for us. We need to remember that data in itself is not magic. Just like my Netflix recommendation algorithm was dependent on my (previously) limited engagements on the platform, our data outputs will only be as dynamic as the information we give it. It is not perfect, either. It (data) is merely a recorded value — by a person or a device — in this world.
Our complex and imperfect world.
Learning to form a healthy relationship with data is, therefore, a necessity to building equity. Because that establishes our commitment to count right and our patterns of engagement with data. To be counted in that data should not be a matter of power or privilege for anyone.
It must be fundamental.
[/et_pb_text][/et_pb_column][/et_pb_row]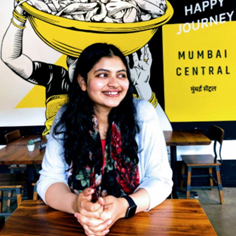
Meenakshi Das
Meenakshi (Meena) Das (she/her/hers) is the founder, data consultant, trainer, and an ethicist with her nonprofit consulting practice, NamasteData. She specializes in designing and teaching equitable research tools and analyzing engagement. Her two workshops referenced in the article are: Advancing Equitable Data Collection and Advancing Equity through Visualizations. Meena appreciates spending her time outside work as a mentor to immigrants. Her recent favorite project is talking about IDEA-led data and research through her LinkedIn-based newsletter data uncollected.
This question is primarily for two reasons:
- I want my co-learners to actively reflect on their lived and learned experiences, so they realize how their (and their community’s collective) privileges and challenges are counted or eliminated from the data, and
- I want each of us to remember and be able to distinguish between “IDEA data” and “IDEA-led data.”“IDEA data” is a clear term — it is the data about inclusion, diversity, equity, and accessibility. “IDEA-led data,” on the contrary, is an evolving term — it is about the usage of data in a way that is grounded in the intentions of inclusion, diversity, equity, and accessibility. This usage of data can be in any shape —collection, analysis, strategy development, etc. “IDEA data” can be seen as a subset of “IDEA-led data.”
I must admit that I deeply appreciate the responses we generate as a group in this process. Each response is unique, and yet, all of them together gives “equity” in data a meaning.
See it this way, ask every household member to clean the messiest room and observe how they do it. I can guarantee they won’t have the same starting point in cleaning that room. Some will start with folding laundry; others might start with picking out all empty bottles, cans, or products in the room. That’s what it is like to learn advancing equity in data. That journey is unique and personal — and it starts with learning about diversity, access, and voice. There is no one checklist of “how-to” here. It starts with asking ourselves — what is our purpose and intention with the data? Does it perpetuate harm or create opportunities of equity and inclusion?
2. Identity is very personal (and fluid at times) information. There is no one right way to capture it, except that it has to be human.
Acknowledge that this is personal and fluid information (fluid because identity information can change). That means it should be a non-negotiable priority to design the questions in a way that centers the people responding to those questions in your data collection exercise.
For example, the questions around gender, race, and sexual orientation are often offered in limited 5-7 checkbox options. Identity is extremely personal information about someone, and 5-7 options may not cover everyone’s personal identity.
There is missing and broken trust in how we have collected and counted data in the past. And, designing those questions, like always, does not generate confidence. After all, it is something for which people continue to be excluded and harmed. Designing data collection questions around identity information thus starts with two fundamentals. One, as the data collection team, being clear about the purpose of the data that is collected. That purpose has to connect with a concrete action. Example: pronouns are asked so they can be used to appropriately acknowledge individuals in written/verbal communication. Two, as designers, being flexible in the way questions are asked so it prioritizes the people who respond. Example: checkbox vs. open-ended identity-based questions. I acknowledge that this second point (being flexible in design) can be tricky at times, and it is something I get to brainstorm with my co-learners.
3. The same data may tell very different stories to each group of people it impacts.
Many years ago, my statistics professor taught us a trick. “Add a quantitative data point and you will generate a reaction.” Years later, I got to use it in my project (and now in this example). Here is an anecdote that I often use and discuss in my workshop:
I am working on a research project that involves evaluating if and how the needs of first-generation immigrants across different generations have changed and shaped the local landscape. That project demands both creativity and patience in data collection (it’s an ongoing[MD1] project). It has everything in the mix – interviews, focus groups, and community surveys.
One of the common questions asked in the interviews and focus groups conducted so far has been, “In 2017, a report published 8 out of 10 employers admit ‘regional accents influence recruitment decisions.’ Do you think accents influence job opportunities as first-generation immigrants?” The response is interesting (on the basis of what is collected as of now). There is a clear difference in the response (verbal and body language) when asked to white, native English speakers vs. non-white, multilingual speakers. Similarly, there is a difference in the response when the respondents were first-generation immigrants across the 70s vs 90s vs 2000s, etc.
Clearly, the same question generates different reactions (and data points).
What I bring back from that example into my workshop discussion is – being mindful about the reports that come out of such data collection instances when there are many different voices in the play. When interpreting and translating that data into insights, it is critical to include:
What is the context here, so we understand qualitative vs. quantitative is better?
- Who collected this data?
- Are there actions on the part of the sponsors in ethical use, distribution, and next steps from this data?
- How was this data collected?
- Who will the insights speak to?
When working with data, there is no specific step where equity needs to be centered; equity should be embedded in the entire process. The point of this conversation is to bring awareness and responsibility to our actions for and from our data.
4. Power is deeply intertwined with language.
Changing the data culture to use data responsibly comes with its roadblocks. Several challenges can surface depending on who is leading those efforts in the organization. Some specific roadblocks could be an inability to get before the right stakeholders, being gaslighted by the right stakeholders, or being shut down by someone who holds more power.
For example, one of the workshop participants once shared how she wanted to update what is collected and reported around the identity of donors, volunteers, and other key groups in her organization. She realized that updates required conversations within and outside her team, so a clear why, what, and how could be collectively established in making those updates. So, she asked for those meetings and space in meeting agendas. But, as an entry-level data coordinator (and a Black woman), she shared how she found resistance to those needed conversations. The updates are still pending and live in an internal tracker.
Each of us is responsible for building a better individual relationship with data. This will look different from role to role. A data analyst will require a different level of understanding in cleaning and analyzing data than a team leader responsible to synthesize a bulky report for the Board. Each will require a different lens on the summarized charts and language of its narratives.
Regardless of how that individual relationship (with data) looks like, the underlying intention here goes back to the first point — reflecting (and acting) on what equity in data means. And, when we make an active effort in understanding “data” and its nuances, we can acknowledge the positional power we have in influencing our data culture.
5. All of us who work in the sector are, to a certain extent, storytellers.
We tell stories using the data we have, for the data we want to collect. We can make those stories inclusive by creating a space of collaboration and feedback with the community whose stories they are while learning to say “I don’t know” at appropriate times.
I particularly love a relevant example I received in the workshop. One of the participants was both an annual-giving fundraiser and the data person in the fundraising team of an independent school. Their mission was to offer learning and development opportunities to kids and youth of low-income families with one or both BIPOC parents. She shared how the impact stories they collected in their data didn’t feel adequate in light of the pandemic challenges, so they changed their process. Instead of looking at their own previously collected data, they opened up the process to include their entire community (families, kids, digital supporters, etc.). Not only did they bring representatives of the community in designing the questions of data collection, but they also supported in summarizing and interpreting the responses to define “impact.”
Remember, the “impact” of the stories is not through the numbers but the people in those stories. In the example above, a fair share of voices were included, right from the way data was collected to how it translated into impact. Let us lean on both the data and the people underlying it.
You and I live in a time when data is constantly and continuously collected about us, through us, claiming it is for us. We need to remember that data in itself is not magic. Just like my Netflix recommendation algorithm was dependent on my (previously) limited engagements on the platform, our data outputs will only be as dynamic as the information we give it. It is not perfect, either. It (data) is merely a recorded value — by a person or a device — in this world.
Our complex and imperfect world.
Learning to form a healthy relationship with data is, therefore, a necessity to building equity. Because that establishes our commitment to count right and our patterns of engagement with data. To be counted in that data should not be a matter of power or privilege for anyone.
It must be fundamental.
[/et_pb_text][/et_pb_column][/et_pb_row]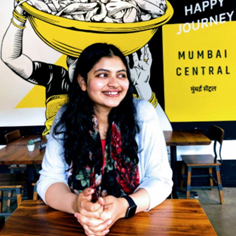
Meenakshi Das
Meenakshi (Meena) Das (she/her/hers) is the founder, data consultant, trainer, and an ethicist with her nonprofit consulting practice, NamasteData. She specializes in designing and teaching equitable research tools and analyzing engagement. Her two workshops referenced in the article are: Advancing Equitable Data Collection and Advancing Equity through Visualizations. Meena appreciates spending her time outside work as a mentor to immigrants. Her recent favorite project is talking about IDEA-led data and research through her LinkedIn-based newsletter data uncollected.
1. What constitutes “equity” in data results from evolving learning around diversity, access, and voice.
I start the workshop with a basic question — “what does IDEA-led data mean to you?” (IDEA refers to Inclusion, Diversity, Equity, and Accessibility)
This question is primarily for two reasons:
- I want my co-learners to actively reflect on their lived and learned experiences, so they realize how their (and their community’s collective) privileges and challenges are counted or eliminated from the data, and
- I want each of us to remember and be able to distinguish between “IDEA data” and “IDEA-led data.”“IDEA data” is a clear term — it is the data about inclusion, diversity, equity, and accessibility. “IDEA-led data,” on the contrary, is an evolving term — it is about the usage of data in a way that is grounded in the intentions of inclusion, diversity, equity, and accessibility. This usage of data can be in any shape —collection, analysis, strategy development, etc. “IDEA data” can be seen as a subset of “IDEA-led data.”
I must admit that I deeply appreciate the responses we generate as a group in this process. Each response is unique, and yet, all of them together gives “equity” in data a meaning.
See it this way, ask every household member to clean the messiest room and observe how they do it. I can guarantee they won’t have the same starting point in cleaning that room. Some will start with folding laundry; others might start with picking out all empty bottles, cans, or products in the room. That’s what it is like to learn advancing equity in data. That journey is unique and personal — and it starts with learning about diversity, access, and voice. There is no one checklist of “how-to” here. It starts with asking ourselves — what is our purpose and intention with the data? Does it perpetuate harm or create opportunities of equity and inclusion?
2. Identity is very personal (and fluid at times) information. There is no one right way to capture it, except that it has to be human.
Acknowledge that this is personal and fluid information (fluid because identity information can change). That means it should be a non-negotiable priority to design the questions in a way that centers the people responding to those questions in your data collection exercise.
For example, the questions around gender, race, and sexual orientation are often offered in limited 5-7 checkbox options. Identity is extremely personal information about someone, and 5-7 options may not cover everyone’s personal identity.
There is missing and broken trust in how we have collected and counted data in the past. And, designing those questions, like always, does not generate confidence. After all, it is something for which people continue to be excluded and harmed. Designing data collection questions around identity information thus starts with two fundamentals. One, as the data collection team, being clear about the purpose of the data that is collected. That purpose has to connect with a concrete action. Example: pronouns are asked so they can be used to appropriately acknowledge individuals in written/verbal communication. Two, as designers, being flexible in the way questions are asked so it prioritizes the people who respond. Example: checkbox vs. open-ended identity-based questions. I acknowledge that this second point (being flexible in design) can be tricky at times, and it is something I get to brainstorm with my co-learners.
3. The same data may tell very different stories to each group of people it impacts.
Many years ago, my statistics professor taught us a trick. “Add a quantitative data point and you will generate a reaction.” Years later, I got to use it in my project (and now in this example). Here is an anecdote that I often use and discuss in my workshop:
I am working on a research project that involves evaluating if and how the needs of first-generation immigrants across different generations have changed and shaped the local landscape. That project demands both creativity and patience in data collection (it’s an ongoing[MD1] project). It has everything in the mix – interviews, focus groups, and community surveys.
One of the common questions asked in the interviews and focus groups conducted so far has been, “In 2017, a report published 8 out of 10 employers admit ‘regional accents influence recruitment decisions.’ Do you think accents influence job opportunities as first-generation immigrants?” The response is interesting (on the basis of what is collected as of now). There is a clear difference in the response (verbal and body language) when asked to white, native English speakers vs. non-white, multilingual speakers. Similarly, there is a difference in the response when the respondents were first-generation immigrants across the 70s vs 90s vs 2000s, etc.
Clearly, the same question generates different reactions (and data points).
What I bring back from that example into my workshop discussion is – being mindful about the reports that come out of such data collection instances when there are many different voices in the play. When interpreting and translating that data into insights, it is critical to include:
What is the context here, so we understand qualitative vs. quantitative is better?
- Who collected this data?
- Are there actions on the part of the sponsors in ethical use, distribution, and next steps from this data?
- How was this data collected?
- Who will the insights speak to?
When working with data, there is no specific step where equity needs to be centered; equity should be embedded in the entire process. The point of this conversation is to bring awareness and responsibility to our actions for and from our data.
4. Power is deeply intertwined with language.
Changing the data culture to use data responsibly comes with its roadblocks. Several challenges can surface depending on who is leading those efforts in the organization. Some specific roadblocks could be an inability to get before the right stakeholders, being gaslighted by the right stakeholders, or being shut down by someone who holds more power.
For example, one of the workshop participants once shared how she wanted to update what is collected and reported around the identity of donors, volunteers, and other key groups in her organization. She realized that updates required conversations within and outside her team, so a clear why, what, and how could be collectively established in making those updates. So, she asked for those meetings and space in meeting agendas. But, as an entry-level data coordinator (and a Black woman), she shared how she found resistance to those needed conversations. The updates are still pending and live in an internal tracker.
Each of us is responsible for building a better individual relationship with data. This will look different from role to role. A data analyst will require a different level of understanding in cleaning and analyzing data than a team leader responsible to synthesize a bulky report for the Board. Each will require a different lens on the summarized charts and language of its narratives.
Regardless of how that individual relationship (with data) looks like, the underlying intention here goes back to the first point — reflecting (and acting) on what equity in data means. And, when we make an active effort in understanding “data” and its nuances, we can acknowledge the positional power we have in influencing our data culture.
5. All of us who work in the sector are, to a certain extent, storytellers.
We tell stories using the data we have, for the data we want to collect. We can make those stories inclusive by creating a space of collaboration and feedback with the community whose stories they are while learning to say “I don’t know” at appropriate times.
I particularly love a relevant example I received in the workshop. One of the participants was both an annual-giving fundraiser and the data person in the fundraising team of an independent school. Their mission was to offer learning and development opportunities to kids and youth of low-income families with one or both BIPOC parents. She shared how the impact stories they collected in their data didn’t feel adequate in light of the pandemic challenges, so they changed their process. Instead of looking at their own previously collected data, they opened up the process to include their entire community (families, kids, digital supporters, etc.). Not only did they bring representatives of the community in designing the questions of data collection, but they also supported in summarizing and interpreting the responses to define “impact.”
Remember, the “impact” of the stories is not through the numbers but the people in those stories. In the example above, a fair share of voices were included, right from the way data was collected to how it translated into impact. Let us lean on both the data and the people underlying it.
You and I live in a time when data is constantly and continuously collected about us, through us, claiming it is for us. We need to remember that data in itself is not magic. Just like my Netflix recommendation algorithm was dependent on my (previously) limited engagements on the platform, our data outputs will only be as dynamic as the information we give it. It is not perfect, either. It (data) is merely a recorded value — by a person or a device — in this world.
Our complex and imperfect world.
Learning to form a healthy relationship with data is, therefore, a necessity to building equity. Because that establishes our commitment to count right and our patterns of engagement with data. To be counted in that data should not be a matter of power or privilege for anyone.
It must be fundamental.
[/et_pb_text][/et_pb_column][/et_pb_row]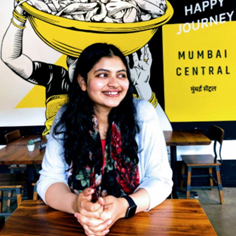
Meenakshi Das
Meenakshi (Meena) Das (she/her/hers) is the founder, data consultant, trainer, and an ethicist with her nonprofit consulting practice, NamasteData. She specializes in designing and teaching equitable research tools and analyzing engagement. Her two workshops referenced in the article are: Advancing Equitable Data Collection and Advancing Equity through Visualizations. Meena appreciates spending her time outside work as a mentor to immigrants. Her recent favorite project is talking about IDEA-led data and research through her LinkedIn-based newsletter data uncollected.
I learned five lessons by offering these workshops to individuals from different roles, sectors, and data comfort that I want to share with you. I call those individuals my co-learners.
1. What constitutes “equity” in data results from evolving learning around diversity, access, and voice.
I start the workshop with a basic question — “what does IDEA-led data mean to you?” (IDEA refers to Inclusion, Diversity, Equity, and Accessibility)
This question is primarily for two reasons:
- I want my co-learners to actively reflect on their lived and learned experiences, so they realize how their (and their community’s collective) privileges and challenges are counted or eliminated from the data, and
- I want each of us to remember and be able to distinguish between “IDEA data” and “IDEA-led data.”“IDEA data” is a clear term — it is the data about inclusion, diversity, equity, and accessibility. “IDEA-led data,” on the contrary, is an evolving term — it is about the usage of data in a way that is grounded in the intentions of inclusion, diversity, equity, and accessibility. This usage of data can be in any shape —collection, analysis, strategy development, etc. “IDEA data” can be seen as a subset of “IDEA-led data.”
I must admit that I deeply appreciate the responses we generate as a group in this process. Each response is unique, and yet, all of them together gives “equity” in data a meaning.
See it this way, ask every household member to clean the messiest room and observe how they do it. I can guarantee they won’t have the same starting point in cleaning that room. Some will start with folding laundry; others might start with picking out all empty bottles, cans, or products in the room. That’s what it is like to learn advancing equity in data. That journey is unique and personal — and it starts with learning about diversity, access, and voice. There is no one checklist of “how-to” here. It starts with asking ourselves — what is our purpose and intention with the data? Does it perpetuate harm or create opportunities of equity and inclusion?
2. Identity is very personal (and fluid at times) information. There is no one right way to capture it, except that it has to be human.
Acknowledge that this is personal and fluid information (fluid because identity information can change). That means it should be a non-negotiable priority to design the questions in a way that centers the people responding to those questions in your data collection exercise.
For example, the questions around gender, race, and sexual orientation are often offered in limited 5-7 checkbox options. Identity is extremely personal information about someone, and 5-7 options may not cover everyone’s personal identity.
There is missing and broken trust in how we have collected and counted data in the past. And, designing those questions, like always, does not generate confidence. After all, it is something for which people continue to be excluded and harmed. Designing data collection questions around identity information thus starts with two fundamentals. One, as the data collection team, being clear about the purpose of the data that is collected. That purpose has to connect with a concrete action. Example: pronouns are asked so they can be used to appropriately acknowledge individuals in written/verbal communication. Two, as designers, being flexible in the way questions are asked so it prioritizes the people who respond. Example: checkbox vs. open-ended identity-based questions. I acknowledge that this second point (being flexible in design) can be tricky at times, and it is something I get to brainstorm with my co-learners.
3. The same data may tell very different stories to each group of people it impacts.
Many years ago, my statistics professor taught us a trick. “Add a quantitative data point and you will generate a reaction.” Years later, I got to use it in my project (and now in this example). Here is an anecdote that I often use and discuss in my workshop:
I am working on a research project that involves evaluating if and how the needs of first-generation immigrants across different generations have changed and shaped the local landscape. That project demands both creativity and patience in data collection (it’s an ongoing[MD1] project). It has everything in the mix – interviews, focus groups, and community surveys.
One of the common questions asked in the interviews and focus groups conducted so far has been, “In 2017, a report published 8 out of 10 employers admit ‘regional accents influence recruitment decisions.’ Do you think accents influence job opportunities as first-generation immigrants?” The response is interesting (on the basis of what is collected as of now). There is a clear difference in the response (verbal and body language) when asked to white, native English speakers vs. non-white, multilingual speakers. Similarly, there is a difference in the response when the respondents were first-generation immigrants across the 70s vs 90s vs 2000s, etc.
Clearly, the same question generates different reactions (and data points).
What I bring back from that example into my workshop discussion is – being mindful about the reports that come out of such data collection instances when there are many different voices in the play. When interpreting and translating that data into insights, it is critical to include:
What is the context here, so we understand qualitative vs. quantitative is better?
- Who collected this data?
- Are there actions on the part of the sponsors in ethical use, distribution, and next steps from this data?
- How was this data collected?
- Who will the insights speak to?
When working with data, there is no specific step where equity needs to be centered; equity should be embedded in the entire process. The point of this conversation is to bring awareness and responsibility to our actions for and from our data.
4. Power is deeply intertwined with language.
Changing the data culture to use data responsibly comes with its roadblocks. Several challenges can surface depending on who is leading those efforts in the organization. Some specific roadblocks could be an inability to get before the right stakeholders, being gaslighted by the right stakeholders, or being shut down by someone who holds more power.
For example, one of the workshop participants once shared how she wanted to update what is collected and reported around the identity of donors, volunteers, and other key groups in her organization. She realized that updates required conversations within and outside her team, so a clear why, what, and how could be collectively established in making those updates. So, she asked for those meetings and space in meeting agendas. But, as an entry-level data coordinator (and a Black woman), she shared how she found resistance to those needed conversations. The updates are still pending and live in an internal tracker.
Each of us is responsible for building a better individual relationship with data. This will look different from role to role. A data analyst will require a different level of understanding in cleaning and analyzing data than a team leader responsible to synthesize a bulky report for the Board. Each will require a different lens on the summarized charts and language of its narratives.
Regardless of how that individual relationship (with data) looks like, the underlying intention here goes back to the first point — reflecting (and acting) on what equity in data means. And, when we make an active effort in understanding “data” and its nuances, we can acknowledge the positional power we have in influencing our data culture.
5. All of us who work in the sector are, to a certain extent, storytellers.
We tell stories using the data we have, for the data we want to collect. We can make those stories inclusive by creating a space of collaboration and feedback with the community whose stories they are while learning to say “I don’t know” at appropriate times.
I particularly love a relevant example I received in the workshop. One of the participants was both an annual-giving fundraiser and the data person in the fundraising team of an independent school. Their mission was to offer learning and development opportunities to kids and youth of low-income families with one or both BIPOC parents. She shared how the impact stories they collected in their data didn’t feel adequate in light of the pandemic challenges, so they changed their process. Instead of looking at their own previously collected data, they opened up the process to include their entire community (families, kids, digital supporters, etc.). Not only did they bring representatives of the community in designing the questions of data collection, but they also supported in summarizing and interpreting the responses to define “impact.”
Remember, the “impact” of the stories is not through the numbers but the people in those stories. In the example above, a fair share of voices were included, right from the way data was collected to how it translated into impact. Let us lean on both the data and the people underlying it.
You and I live in a time when data is constantly and continuously collected about us, through us, claiming it is for us. We need to remember that data in itself is not magic. Just like my Netflix recommendation algorithm was dependent on my (previously) limited engagements on the platform, our data outputs will only be as dynamic as the information we give it. It is not perfect, either. It (data) is merely a recorded value — by a person or a device — in this world.
Our complex and imperfect world.
Learning to form a healthy relationship with data is, therefore, a necessity to building equity. Because that establishes our commitment to count right and our patterns of engagement with data. To be counted in that data should not be a matter of power or privilege for anyone.
It must be fundamental.
[/et_pb_text][/et_pb_column][/et_pb_row]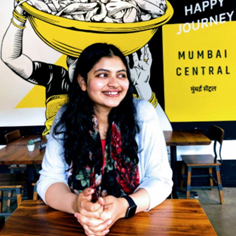
Meenakshi Das
Meenakshi (Meena) Das (she/her/hers) is the founder, data consultant, trainer, and an ethicist with her nonprofit consulting practice, NamasteData. She specializes in designing and teaching equitable research tools and analyzing engagement. Her two workshops referenced in the article are: Advancing Equitable Data Collection and Advancing Equity through Visualizations. Meena appreciates spending her time outside work as a mentor to immigrants. Her recent favorite project is talking about IDEA-led data and research through her LinkedIn-based newsletter data uncollected.
My workshops to advance equity in data are built with this intention — to build collective knowledge around data collection and visualization in a way that allows us to appropriately challenge those places where data can lead to exclusion and alienation.
I learned five lessons by offering these workshops to individuals from different roles, sectors, and data comfort that I want to share with you. I call those individuals my co-learners.
1. What constitutes “equity” in data results from evolving learning around diversity, access, and voice.
I start the workshop with a basic question — “what does IDEA-led data mean to you?” (IDEA refers to Inclusion, Diversity, Equity, and Accessibility)
This question is primarily for two reasons:
- I want my co-learners to actively reflect on their lived and learned experiences, so they realize how their (and their community’s collective) privileges and challenges are counted or eliminated from the data, and
- I want each of us to remember and be able to distinguish between “IDEA data” and “IDEA-led data.”“IDEA data” is a clear term — it is the data about inclusion, diversity, equity, and accessibility. “IDEA-led data,” on the contrary, is an evolving term — it is about the usage of data in a way that is grounded in the intentions of inclusion, diversity, equity, and accessibility. This usage of data can be in any shape —collection, analysis, strategy development, etc. “IDEA data” can be seen as a subset of “IDEA-led data.”
I must admit that I deeply appreciate the responses we generate as a group in this process. Each response is unique, and yet, all of them together gives “equity” in data a meaning.
See it this way, ask every household member to clean the messiest room and observe how they do it. I can guarantee they won’t have the same starting point in cleaning that room. Some will start with folding laundry; others might start with picking out all empty bottles, cans, or products in the room. That’s what it is like to learn advancing equity in data. That journey is unique and personal — and it starts with learning about diversity, access, and voice. There is no one checklist of “how-to” here. It starts with asking ourselves — what is our purpose and intention with the data? Does it perpetuate harm or create opportunities of equity and inclusion?
2. Identity is very personal (and fluid at times) information. There is no one right way to capture it, except that it has to be human.
Acknowledge that this is personal and fluid information (fluid because identity information can change). That means it should be a non-negotiable priority to design the questions in a way that centers the people responding to those questions in your data collection exercise.
For example, the questions around gender, race, and sexual orientation are often offered in limited 5-7 checkbox options. Identity is extremely personal information about someone, and 5-7 options may not cover everyone’s personal identity.
There is missing and broken trust in how we have collected and counted data in the past. And, designing those questions, like always, does not generate confidence. After all, it is something for which people continue to be excluded and harmed. Designing data collection questions around identity information thus starts with two fundamentals. One, as the data collection team, being clear about the purpose of the data that is collected. That purpose has to connect with a concrete action. Example: pronouns are asked so they can be used to appropriately acknowledge individuals in written/verbal communication. Two, as designers, being flexible in the way questions are asked so it prioritizes the people who respond. Example: checkbox vs. open-ended identity-based questions. I acknowledge that this second point (being flexible in design) can be tricky at times, and it is something I get to brainstorm with my co-learners.
3. The same data may tell very different stories to each group of people it impacts.
Many years ago, my statistics professor taught us a trick. “Add a quantitative data point and you will generate a reaction.” Years later, I got to use it in my project (and now in this example). Here is an anecdote that I often use and discuss in my workshop:
I am working on a research project that involves evaluating if and how the needs of first-generation immigrants across different generations have changed and shaped the local landscape. That project demands both creativity and patience in data collection (it’s an ongoing[MD1] project). It has everything in the mix – interviews, focus groups, and community surveys.
One of the common questions asked in the interviews and focus groups conducted so far has been, “In 2017, a report published 8 out of 10 employers admit ‘regional accents influence recruitment decisions.’ Do you think accents influence job opportunities as first-generation immigrants?” The response is interesting (on the basis of what is collected as of now). There is a clear difference in the response (verbal and body language) when asked to white, native English speakers vs. non-white, multilingual speakers. Similarly, there is a difference in the response when the respondents were first-generation immigrants across the 70s vs 90s vs 2000s, etc.
Clearly, the same question generates different reactions (and data points).
What I bring back from that example into my workshop discussion is – being mindful about the reports that come out of such data collection instances when there are many different voices in the play. When interpreting and translating that data into insights, it is critical to include:
What is the context here, so we understand qualitative vs. quantitative is better?
- Who collected this data?
- Are there actions on the part of the sponsors in ethical use, distribution, and next steps from this data?
- How was this data collected?
- Who will the insights speak to?
When working with data, there is no specific step where equity needs to be centered; equity should be embedded in the entire process. The point of this conversation is to bring awareness and responsibility to our actions for and from our data.
4. Power is deeply intertwined with language.
Changing the data culture to use data responsibly comes with its roadblocks. Several challenges can surface depending on who is leading those efforts in the organization. Some specific roadblocks could be an inability to get before the right stakeholders, being gaslighted by the right stakeholders, or being shut down by someone who holds more power.
For example, one of the workshop participants once shared how she wanted to update what is collected and reported around the identity of donors, volunteers, and other key groups in her organization. She realized that updates required conversations within and outside her team, so a clear why, what, and how could be collectively established in making those updates. So, she asked for those meetings and space in meeting agendas. But, as an entry-level data coordinator (and a Black woman), she shared how she found resistance to those needed conversations. The updates are still pending and live in an internal tracker.
Each of us is responsible for building a better individual relationship with data. This will look different from role to role. A data analyst will require a different level of understanding in cleaning and analyzing data than a team leader responsible to synthesize a bulky report for the Board. Each will require a different lens on the summarized charts and language of its narratives.
Regardless of how that individual relationship (with data) looks like, the underlying intention here goes back to the first point — reflecting (and acting) on what equity in data means. And, when we make an active effort in understanding “data” and its nuances, we can acknowledge the positional power we have in influencing our data culture.
5. All of us who work in the sector are, to a certain extent, storytellers.
We tell stories using the data we have, for the data we want to collect. We can make those stories inclusive by creating a space of collaboration and feedback with the community whose stories they are while learning to say “I don’t know” at appropriate times.
I particularly love a relevant example I received in the workshop. One of the participants was both an annual-giving fundraiser and the data person in the fundraising team of an independent school. Their mission was to offer learning and development opportunities to kids and youth of low-income families with one or both BIPOC parents. She shared how the impact stories they collected in their data didn’t feel adequate in light of the pandemic challenges, so they changed their process. Instead of looking at their own previously collected data, they opened up the process to include their entire community (families, kids, digital supporters, etc.). Not only did they bring representatives of the community in designing the questions of data collection, but they also supported in summarizing and interpreting the responses to define “impact.”
Remember, the “impact” of the stories is not through the numbers but the people in those stories. In the example above, a fair share of voices were included, right from the way data was collected to how it translated into impact. Let us lean on both the data and the people underlying it.
You and I live in a time when data is constantly and continuously collected about us, through us, claiming it is for us. We need to remember that data in itself is not magic. Just like my Netflix recommendation algorithm was dependent on my (previously) limited engagements on the platform, our data outputs will only be as dynamic as the information we give it. It is not perfect, either. It (data) is merely a recorded value — by a person or a device — in this world.
Our complex and imperfect world.
Learning to form a healthy relationship with data is, therefore, a necessity to building equity. Because that establishes our commitment to count right and our patterns of engagement with data. To be counted in that data should not be a matter of power or privilege for anyone.
It must be fundamental.
[/et_pb_text][/et_pb_column][/et_pb_row]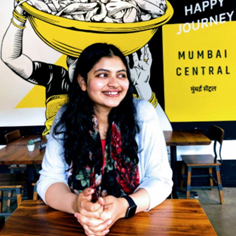
Meenakshi Das
Meenakshi (Meena) Das (she/her/hers) is the founder, data consultant, trainer, and an ethicist with her nonprofit consulting practice, NamasteData. She specializes in designing and teaching equitable research tools and analyzing engagement. Her two workshops referenced in the article are: Advancing Equitable Data Collection and Advancing Equity through Visualizations. Meena appreciates spending her time outside work as a mentor to immigrants. Her recent favorite project is talking about IDEA-led data and research through her LinkedIn-based newsletter data uncollected.
Here is the danger of extreme personalization — we risk limiting ourselves to what we know, what we understand, and what makes us comfortable. After “confusing” Netflix’s recommendation algorithm several times, I have learned about horses, street-food business women in South America, the story behind the thickness of Thai noodles, and, of course, more Korean and Turkish dramas.
My workshops to advance equity in data are built with this intention — to build collective knowledge around data collection and visualization in a way that allows us to appropriately challenge those places where data can lead to exclusion and alienation.
I learned five lessons by offering these workshops to individuals from different roles, sectors, and data comfort that I want to share with you. I call those individuals my co-learners.
1. What constitutes “equity” in data results from evolving learning around diversity, access, and voice.
I start the workshop with a basic question — “what does IDEA-led data mean to you?” (IDEA refers to Inclusion, Diversity, Equity, and Accessibility)
This question is primarily for two reasons:
- I want my co-learners to actively reflect on their lived and learned experiences, so they realize how their (and their community’s collective) privileges and challenges are counted or eliminated from the data, and
- I want each of us to remember and be able to distinguish between “IDEA data” and “IDEA-led data.”“IDEA data” is a clear term — it is the data about inclusion, diversity, equity, and accessibility. “IDEA-led data,” on the contrary, is an evolving term — it is about the usage of data in a way that is grounded in the intentions of inclusion, diversity, equity, and accessibility. This usage of data can be in any shape —collection, analysis, strategy development, etc. “IDEA data” can be seen as a subset of “IDEA-led data.”
I must admit that I deeply appreciate the responses we generate as a group in this process. Each response is unique, and yet, all of them together gives “equity” in data a meaning.
See it this way, ask every household member to clean the messiest room and observe how they do it. I can guarantee they won’t have the same starting point in cleaning that room. Some will start with folding laundry; others might start with picking out all empty bottles, cans, or products in the room. That’s what it is like to learn advancing equity in data. That journey is unique and personal — and it starts with learning about diversity, access, and voice. There is no one checklist of “how-to” here. It starts with asking ourselves — what is our purpose and intention with the data? Does it perpetuate harm or create opportunities of equity and inclusion?
2. Identity is very personal (and fluid at times) information. There is no one right way to capture it, except that it has to be human.
Acknowledge that this is personal and fluid information (fluid because identity information can change). That means it should be a non-negotiable priority to design the questions in a way that centers the people responding to those questions in your data collection exercise.
For example, the questions around gender, race, and sexual orientation are often offered in limited 5-7 checkbox options. Identity is extremely personal information about someone, and 5-7 options may not cover everyone’s personal identity.
There is missing and broken trust in how we have collected and counted data in the past. And, designing those questions, like always, does not generate confidence. After all, it is something for which people continue to be excluded and harmed. Designing data collection questions around identity information thus starts with two fundamentals. One, as the data collection team, being clear about the purpose of the data that is collected. That purpose has to connect with a concrete action. Example: pronouns are asked so they can be used to appropriately acknowledge individuals in written/verbal communication. Two, as designers, being flexible in the way questions are asked so it prioritizes the people who respond. Example: checkbox vs. open-ended identity-based questions. I acknowledge that this second point (being flexible in design) can be tricky at times, and it is something I get to brainstorm with my co-learners.
3. The same data may tell very different stories to each group of people it impacts.
Many years ago, my statistics professor taught us a trick. “Add a quantitative data point and you will generate a reaction.” Years later, I got to use it in my project (and now in this example). Here is an anecdote that I often use and discuss in my workshop:
I am working on a research project that involves evaluating if and how the needs of first-generation immigrants across different generations have changed and shaped the local landscape. That project demands both creativity and patience in data collection (it’s an ongoing[MD1] project). It has everything in the mix – interviews, focus groups, and community surveys.
One of the common questions asked in the interviews and focus groups conducted so far has been, “In 2017, a report published 8 out of 10 employers admit ‘regional accents influence recruitment decisions.’ Do you think accents influence job opportunities as first-generation immigrants?” The response is interesting (on the basis of what is collected as of now). There is a clear difference in the response (verbal and body language) when asked to white, native English speakers vs. non-white, multilingual speakers. Similarly, there is a difference in the response when the respondents were first-generation immigrants across the 70s vs 90s vs 2000s, etc.
Clearly, the same question generates different reactions (and data points).
What I bring back from that example into my workshop discussion is – being mindful about the reports that come out of such data collection instances when there are many different voices in the play. When interpreting and translating that data into insights, it is critical to include:
What is the context here, so we understand qualitative vs. quantitative is better?
- Who collected this data?
- Are there actions on the part of the sponsors in ethical use, distribution, and next steps from this data?
- How was this data collected?
- Who will the insights speak to?
When working with data, there is no specific step where equity needs to be centered; equity should be embedded in the entire process. The point of this conversation is to bring awareness and responsibility to our actions for and from our data.
4. Power is deeply intertwined with language.
Changing the data culture to use data responsibly comes with its roadblocks. Several challenges can surface depending on who is leading those efforts in the organization. Some specific roadblocks could be an inability to get before the right stakeholders, being gaslighted by the right stakeholders, or being shut down by someone who holds more power.
For example, one of the workshop participants once shared how she wanted to update what is collected and reported around the identity of donors, volunteers, and other key groups in her organization. She realized that updates required conversations within and outside her team, so a clear why, what, and how could be collectively established in making those updates. So, she asked for those meetings and space in meeting agendas. But, as an entry-level data coordinator (and a Black woman), she shared how she found resistance to those needed conversations. The updates are still pending and live in an internal tracker.
Each of us is responsible for building a better individual relationship with data. This will look different from role to role. A data analyst will require a different level of understanding in cleaning and analyzing data than a team leader responsible to synthesize a bulky report for the Board. Each will require a different lens on the summarized charts and language of its narratives.
Regardless of how that individual relationship (with data) looks like, the underlying intention here goes back to the first point — reflecting (and acting) on what equity in data means. And, when we make an active effort in understanding “data” and its nuances, we can acknowledge the positional power we have in influencing our data culture.
5. All of us who work in the sector are, to a certain extent, storytellers.
We tell stories using the data we have, for the data we want to collect. We can make those stories inclusive by creating a space of collaboration and feedback with the community whose stories they are while learning to say “I don’t know” at appropriate times.
I particularly love a relevant example I received in the workshop. One of the participants was both an annual-giving fundraiser and the data person in the fundraising team of an independent school. Their mission was to offer learning and development opportunities to kids and youth of low-income families with one or both BIPOC parents. She shared how the impact stories they collected in their data didn’t feel adequate in light of the pandemic challenges, so they changed their process. Instead of looking at their own previously collected data, they opened up the process to include their entire community (families, kids, digital supporters, etc.). Not only did they bring representatives of the community in designing the questions of data collection, but they also supported in summarizing and interpreting the responses to define “impact.”
Remember, the “impact” of the stories is not through the numbers but the people in those stories. In the example above, a fair share of voices were included, right from the way data was collected to how it translated into impact. Let us lean on both the data and the people underlying it.
You and I live in a time when data is constantly and continuously collected about us, through us, claiming it is for us. We need to remember that data in itself is not magic. Just like my Netflix recommendation algorithm was dependent on my (previously) limited engagements on the platform, our data outputs will only be as dynamic as the information we give it. It is not perfect, either. It (data) is merely a recorded value — by a person or a device — in this world.
Our complex and imperfect world.
Learning to form a healthy relationship with data is, therefore, a necessity to building equity. Because that establishes our commitment to count right and our patterns of engagement with data. To be counted in that data should not be a matter of power or privilege for anyone.
It must be fundamental.
[/et_pb_text][/et_pb_column][/et_pb_row]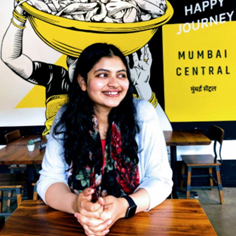
Meenakshi Das
Meenakshi (Meena) Das (she/her/hers) is the founder, data consultant, trainer, and an ethicist with her nonprofit consulting practice, NamasteData. She specializes in designing and teaching equitable research tools and analyzing engagement. Her two workshops referenced in the article are: Advancing Equitable Data Collection and Advancing Equity through Visualizations. Meena appreciates spending her time outside work as a mentor to immigrants. Her recent favorite project is talking about IDEA-led data and research through her LinkedIn-based newsletter data uncollected.
Let me back up a little to expand on that Netflix example: that platform takes pride in the level of personalization and recommendations it can offer its viewers. Until a few years ago, my Netflix “top picks for you” was a list of stories I was comfortable with — Hallmark classics and very specific thrillers. It was not until I deliberately searched and found my first K-Drama that it started to appear on my recommendation list.
Here is the danger of extreme personalization — we risk limiting ourselves to what we know, what we understand, and what makes us comfortable. After “confusing” Netflix’s recommendation algorithm several times, I have learned about horses, street-food business women in South America, the story behind the thickness of Thai noodles, and, of course, more Korean and Turkish dramas.
My workshops to advance equity in data are built with this intention — to build collective knowledge around data collection and visualization in a way that allows us to appropriately challenge those places where data can lead to exclusion and alienation.
I learned five lessons by offering these workshops to individuals from different roles, sectors, and data comfort that I want to share with you. I call those individuals my co-learners.
1. What constitutes “equity” in data results from evolving learning around diversity, access, and voice.
I start the workshop with a basic question — “what does IDEA-led data mean to you?” (IDEA refers to Inclusion, Diversity, Equity, and Accessibility)
This question is primarily for two reasons:
- I want my co-learners to actively reflect on their lived and learned experiences, so they realize how their (and their community’s collective) privileges and challenges are counted or eliminated from the data, and
- I want each of us to remember and be able to distinguish between “IDEA data” and “IDEA-led data.”“IDEA data” is a clear term — it is the data about inclusion, diversity, equity, and accessibility. “IDEA-led data,” on the contrary, is an evolving term — it is about the usage of data in a way that is grounded in the intentions of inclusion, diversity, equity, and accessibility. This usage of data can be in any shape —collection, analysis, strategy development, etc. “IDEA data” can be seen as a subset of “IDEA-led data.”
I must admit that I deeply appreciate the responses we generate as a group in this process. Each response is unique, and yet, all of them together gives “equity” in data a meaning.
See it this way, ask every household member to clean the messiest room and observe how they do it. I can guarantee they won’t have the same starting point in cleaning that room. Some will start with folding laundry; others might start with picking out all empty bottles, cans, or products in the room. That’s what it is like to learn advancing equity in data. That journey is unique and personal — and it starts with learning about diversity, access, and voice. There is no one checklist of “how-to” here. It starts with asking ourselves — what is our purpose and intention with the data? Does it perpetuate harm or create opportunities of equity and inclusion?
2. Identity is very personal (and fluid at times) information. There is no one right way to capture it, except that it has to be human.
Acknowledge that this is personal and fluid information (fluid because identity information can change). That means it should be a non-negotiable priority to design the questions in a way that centers the people responding to those questions in your data collection exercise.
For example, the questions around gender, race, and sexual orientation are often offered in limited 5-7 checkbox options. Identity is extremely personal information about someone, and 5-7 options may not cover everyone’s personal identity.
There is missing and broken trust in how we have collected and counted data in the past. And, designing those questions, like always, does not generate confidence. After all, it is something for which people continue to be excluded and harmed. Designing data collection questions around identity information thus starts with two fundamentals. One, as the data collection team, being clear about the purpose of the data that is collected. That purpose has to connect with a concrete action. Example: pronouns are asked so they can be used to appropriately acknowledge individuals in written/verbal communication. Two, as designers, being flexible in the way questions are asked so it prioritizes the people who respond. Example: checkbox vs. open-ended identity-based questions. I acknowledge that this second point (being flexible in design) can be tricky at times, and it is something I get to brainstorm with my co-learners.
3. The same data may tell very different stories to each group of people it impacts.
Many years ago, my statistics professor taught us a trick. “Add a quantitative data point and you will generate a reaction.” Years later, I got to use it in my project (and now in this example). Here is an anecdote that I often use and discuss in my workshop:
I am working on a research project that involves evaluating if and how the needs of first-generation immigrants across different generations have changed and shaped the local landscape. That project demands both creativity and patience in data collection (it’s an ongoing[MD1] project). It has everything in the mix – interviews, focus groups, and community surveys.
One of the common questions asked in the interviews and focus groups conducted so far has been, “In 2017, a report published 8 out of 10 employers admit ‘regional accents influence recruitment decisions.’ Do you think accents influence job opportunities as first-generation immigrants?” The response is interesting (on the basis of what is collected as of now). There is a clear difference in the response (verbal and body language) when asked to white, native English speakers vs. non-white, multilingual speakers. Similarly, there is a difference in the response when the respondents were first-generation immigrants across the 70s vs 90s vs 2000s, etc.
Clearly, the same question generates different reactions (and data points).
What I bring back from that example into my workshop discussion is – being mindful about the reports that come out of such data collection instances when there are many different voices in the play. When interpreting and translating that data into insights, it is critical to include:
What is the context here, so we understand qualitative vs. quantitative is better?
- Who collected this data?
- Are there actions on the part of the sponsors in ethical use, distribution, and next steps from this data?
- How was this data collected?
- Who will the insights speak to?
When working with data, there is no specific step where equity needs to be centered; equity should be embedded in the entire process. The point of this conversation is to bring awareness and responsibility to our actions for and from our data.
4. Power is deeply intertwined with language.
Changing the data culture to use data responsibly comes with its roadblocks. Several challenges can surface depending on who is leading those efforts in the organization. Some specific roadblocks could be an inability to get before the right stakeholders, being gaslighted by the right stakeholders, or being shut down by someone who holds more power.
For example, one of the workshop participants once shared how she wanted to update what is collected and reported around the identity of donors, volunteers, and other key groups in her organization. She realized that updates required conversations within and outside her team, so a clear why, what, and how could be collectively established in making those updates. So, she asked for those meetings and space in meeting agendas. But, as an entry-level data coordinator (and a Black woman), she shared how she found resistance to those needed conversations. The updates are still pending and live in an internal tracker.
Each of us is responsible for building a better individual relationship with data. This will look different from role to role. A data analyst will require a different level of understanding in cleaning and analyzing data than a team leader responsible to synthesize a bulky report for the Board. Each will require a different lens on the summarized charts and language of its narratives.
Regardless of how that individual relationship (with data) looks like, the underlying intention here goes back to the first point — reflecting (and acting) on what equity in data means. And, when we make an active effort in understanding “data” and its nuances, we can acknowledge the positional power we have in influencing our data culture.
5. All of us who work in the sector are, to a certain extent, storytellers.
We tell stories using the data we have, for the data we want to collect. We can make those stories inclusive by creating a space of collaboration and feedback with the community whose stories they are while learning to say “I don’t know” at appropriate times.
I particularly love a relevant example I received in the workshop. One of the participants was both an annual-giving fundraiser and the data person in the fundraising team of an independent school. Their mission was to offer learning and development opportunities to kids and youth of low-income families with one or both BIPOC parents. She shared how the impact stories they collected in their data didn’t feel adequate in light of the pandemic challenges, so they changed their process. Instead of looking at their own previously collected data, they opened up the process to include their entire community (families, kids, digital supporters, etc.). Not only did they bring representatives of the community in designing the questions of data collection, but they also supported in summarizing and interpreting the responses to define “impact.”
Remember, the “impact” of the stories is not through the numbers but the people in those stories. In the example above, a fair share of voices were included, right from the way data was collected to how it translated into impact. Let us lean on both the data and the people underlying it.
You and I live in a time when data is constantly and continuously collected about us, through us, claiming it is for us. We need to remember that data in itself is not magic. Just like my Netflix recommendation algorithm was dependent on my (previously) limited engagements on the platform, our data outputs will only be as dynamic as the information we give it. It is not perfect, either. It (data) is merely a recorded value — by a person or a device — in this world.
Our complex and imperfect world.
Learning to form a healthy relationship with data is, therefore, a necessity to building equity. Because that establishes our commitment to count right and our patterns of engagement with data. To be counted in that data should not be a matter of power or privilege for anyone.
It must be fundamental.
[/et_pb_text][/et_pb_column][/et_pb_row]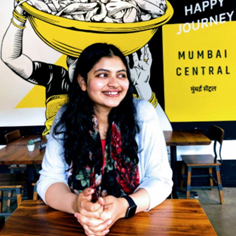
Meenakshi Das
Meenakshi (Meena) Das (she/her/hers) is the founder, data consultant, trainer, and an ethicist with her nonprofit consulting practice, NamasteData. She specializes in designing and teaching equitable research tools and analyzing engagement. Her two workshops referenced in the article are: Advancing Equitable Data Collection and Advancing Equity through Visualizations. Meena appreciates spending her time outside work as a mentor to immigrants. Her recent favorite project is talking about IDEA-led data and research through her LinkedIn-based newsletter data uncollected.
My point here is that it was not until I watched these shows that I realized I must engage with my Netflix account differently to be able to appreciate content beyond what I (and Netflix’s recommendation algorithm) already knew I liked. And that was one of the life lessons that led me to create my two data equity workshops.
Let me back up a little to expand on that Netflix example: that platform takes pride in the level of personalization and recommendations it can offer its viewers. Until a few years ago, my Netflix “top picks for you” was a list of stories I was comfortable with — Hallmark classics and very specific thrillers. It was not until I deliberately searched and found my first K-Drama that it started to appear on my recommendation list.
Here is the danger of extreme personalization — we risk limiting ourselves to what we know, what we understand, and what makes us comfortable. After “confusing” Netflix’s recommendation algorithm several times, I have learned about horses, street-food business women in South America, the story behind the thickness of Thai noodles, and, of course, more Korean and Turkish dramas.
My workshops to advance equity in data are built with this intention — to build collective knowledge around data collection and visualization in a way that allows us to appropriately challenge those places where data can lead to exclusion and alienation.
I learned five lessons by offering these workshops to individuals from different roles, sectors, and data comfort that I want to share with you. I call those individuals my co-learners.
1. What constitutes “equity” in data results from evolving learning around diversity, access, and voice.
I start the workshop with a basic question — “what does IDEA-led data mean to you?” (IDEA refers to Inclusion, Diversity, Equity, and Accessibility)
This question is primarily for two reasons:
- I want my co-learners to actively reflect on their lived and learned experiences, so they realize how their (and their community’s collective) privileges and challenges are counted or eliminated from the data, and
- I want each of us to remember and be able to distinguish between “IDEA data” and “IDEA-led data.”“IDEA data” is a clear term — it is the data about inclusion, diversity, equity, and accessibility. “IDEA-led data,” on the contrary, is an evolving term — it is about the usage of data in a way that is grounded in the intentions of inclusion, diversity, equity, and accessibility. This usage of data can be in any shape —collection, analysis, strategy development, etc. “IDEA data” can be seen as a subset of “IDEA-led data.”
I must admit that I deeply appreciate the responses we generate as a group in this process. Each response is unique, and yet, all of them together gives “equity” in data a meaning.
See it this way, ask every household member to clean the messiest room and observe how they do it. I can guarantee they won’t have the same starting point in cleaning that room. Some will start with folding laundry; others might start with picking out all empty bottles, cans, or products in the room. That’s what it is like to learn advancing equity in data. That journey is unique and personal — and it starts with learning about diversity, access, and voice. There is no one checklist of “how-to” here. It starts with asking ourselves — what is our purpose and intention with the data? Does it perpetuate harm or create opportunities of equity and inclusion?
2. Identity is very personal (and fluid at times) information. There is no one right way to capture it, except that it has to be human.
Acknowledge that this is personal and fluid information (fluid because identity information can change). That means it should be a non-negotiable priority to design the questions in a way that centers the people responding to those questions in your data collection exercise.
For example, the questions around gender, race, and sexual orientation are often offered in limited 5-7 checkbox options. Identity is extremely personal information about someone, and 5-7 options may not cover everyone’s personal identity.
There is missing and broken trust in how we have collected and counted data in the past. And, designing those questions, like always, does not generate confidence. After all, it is something for which people continue to be excluded and harmed. Designing data collection questions around identity information thus starts with two fundamentals. One, as the data collection team, being clear about the purpose of the data that is collected. That purpose has to connect with a concrete action. Example: pronouns are asked so they can be used to appropriately acknowledge individuals in written/verbal communication. Two, as designers, being flexible in the way questions are asked so it prioritizes the people who respond. Example: checkbox vs. open-ended identity-based questions. I acknowledge that this second point (being flexible in design) can be tricky at times, and it is something I get to brainstorm with my co-learners.
3. The same data may tell very different stories to each group of people it impacts.
Many years ago, my statistics professor taught us a trick. “Add a quantitative data point and you will generate a reaction.” Years later, I got to use it in my project (and now in this example). Here is an anecdote that I often use and discuss in my workshop:
I am working on a research project that involves evaluating if and how the needs of first-generation immigrants across different generations have changed and shaped the local landscape. That project demands both creativity and patience in data collection (it’s an ongoing[MD1] project). It has everything in the mix – interviews, focus groups, and community surveys.
One of the common questions asked in the interviews and focus groups conducted so far has been, “In 2017, a report published 8 out of 10 employers admit ‘regional accents influence recruitment decisions.’ Do you think accents influence job opportunities as first-generation immigrants?” The response is interesting (on the basis of what is collected as of now). There is a clear difference in the response (verbal and body language) when asked to white, native English speakers vs. non-white, multilingual speakers. Similarly, there is a difference in the response when the respondents were first-generation immigrants across the 70s vs 90s vs 2000s, etc.
Clearly, the same question generates different reactions (and data points).
What I bring back from that example into my workshop discussion is – being mindful about the reports that come out of such data collection instances when there are many different voices in the play. When interpreting and translating that data into insights, it is critical to include:
What is the context here, so we understand qualitative vs. quantitative is better?
- Who collected this data?
- Are there actions on the part of the sponsors in ethical use, distribution, and next steps from this data?
- How was this data collected?
- Who will the insights speak to?
When working with data, there is no specific step where equity needs to be centered; equity should be embedded in the entire process. The point of this conversation is to bring awareness and responsibility to our actions for and from our data.
4. Power is deeply intertwined with language.
Changing the data culture to use data responsibly comes with its roadblocks. Several challenges can surface depending on who is leading those efforts in the organization. Some specific roadblocks could be an inability to get before the right stakeholders, being gaslighted by the right stakeholders, or being shut down by someone who holds more power.
For example, one of the workshop participants once shared how she wanted to update what is collected and reported around the identity of donors, volunteers, and other key groups in her organization. She realized that updates required conversations within and outside her team, so a clear why, what, and how could be collectively established in making those updates. So, she asked for those meetings and space in meeting agendas. But, as an entry-level data coordinator (and a Black woman), she shared how she found resistance to those needed conversations. The updates are still pending and live in an internal tracker.
Each of us is responsible for building a better individual relationship with data. This will look different from role to role. A data analyst will require a different level of understanding in cleaning and analyzing data than a team leader responsible to synthesize a bulky report for the Board. Each will require a different lens on the summarized charts and language of its narratives.
Regardless of how that individual relationship (with data) looks like, the underlying intention here goes back to the first point — reflecting (and acting) on what equity in data means. And, when we make an active effort in understanding “data” and its nuances, we can acknowledge the positional power we have in influencing our data culture.
5. All of us who work in the sector are, to a certain extent, storytellers.
We tell stories using the data we have, for the data we want to collect. We can make those stories inclusive by creating a space of collaboration and feedback with the community whose stories they are while learning to say “I don’t know” at appropriate times.
I particularly love a relevant example I received in the workshop. One of the participants was both an annual-giving fundraiser and the data person in the fundraising team of an independent school. Their mission was to offer learning and development opportunities to kids and youth of low-income families with one or both BIPOC parents. She shared how the impact stories they collected in their data didn’t feel adequate in light of the pandemic challenges, so they changed their process. Instead of looking at their own previously collected data, they opened up the process to include their entire community (families, kids, digital supporters, etc.). Not only did they bring representatives of the community in designing the questions of data collection, but they also supported in summarizing and interpreting the responses to define “impact.”
Remember, the “impact” of the stories is not through the numbers but the people in those stories. In the example above, a fair share of voices were included, right from the way data was collected to how it translated into impact. Let us lean on both the data and the people underlying it.
You and I live in a time when data is constantly and continuously collected about us, through us, claiming it is for us. We need to remember that data in itself is not magic. Just like my Netflix recommendation algorithm was dependent on my (previously) limited engagements on the platform, our data outputs will only be as dynamic as the information we give it. It is not perfect, either. It (data) is merely a recorded value — by a person or a device — in this world.
Our complex and imperfect world.
Learning to form a healthy relationship with data is, therefore, a necessity to building equity. Because that establishes our commitment to count right and our patterns of engagement with data. To be counted in that data should not be a matter of power or privilege for anyone.
It must be fundamental.
[/et_pb_text][/et_pb_column][/et_pb_row]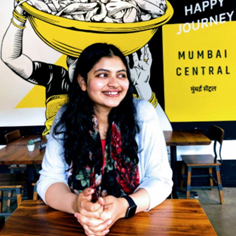
Meenakshi Das
Meenakshi (Meena) Das (she/her/hers) is the founder, data consultant, trainer, and an ethicist with her nonprofit consulting practice, NamasteData. She specializes in designing and teaching equitable research tools and analyzing engagement. Her two workshops referenced in the article are: Advancing Equitable Data Collection and Advancing Equity through Visualizations. Meena appreciates spending her time outside work as a mentor to immigrants. Her recent favorite project is talking about IDEA-led data and research through her LinkedIn-based newsletter data uncollected.
I know this isn’t an essay about TV recommendations (we can do that in our own time).
My point here is that it was not until I watched these shows that I realized I must engage with my Netflix account differently to be able to appreciate content beyond what I (and Netflix’s recommendation algorithm) already knew I liked. And that was one of the life lessons that led me to create my two data equity workshops.
Let me back up a little to expand on that Netflix example: that platform takes pride in the level of personalization and recommendations it can offer its viewers. Until a few years ago, my Netflix “top picks for you” was a list of stories I was comfortable with — Hallmark classics and very specific thrillers. It was not until I deliberately searched and found my first K-Drama that it started to appear on my recommendation list.
Here is the danger of extreme personalization — we risk limiting ourselves to what we know, what we understand, and what makes us comfortable. After “confusing” Netflix’s recommendation algorithm several times, I have learned about horses, street-food business women in South America, the story behind the thickness of Thai noodles, and, of course, more Korean and Turkish dramas.
My workshops to advance equity in data are built with this intention — to build collective knowledge around data collection and visualization in a way that allows us to appropriately challenge those places where data can lead to exclusion and alienation.
I learned five lessons by offering these workshops to individuals from different roles, sectors, and data comfort that I want to share with you. I call those individuals my co-learners.
1. What constitutes “equity” in data results from evolving learning around diversity, access, and voice.
I start the workshop with a basic question — “what does IDEA-led data mean to you?” (IDEA refers to Inclusion, Diversity, Equity, and Accessibility)
This question is primarily for two reasons:
- I want my co-learners to actively reflect on their lived and learned experiences, so they realize how their (and their community’s collective) privileges and challenges are counted or eliminated from the data, and
- I want each of us to remember and be able to distinguish between “IDEA data” and “IDEA-led data.”“IDEA data” is a clear term — it is the data about inclusion, diversity, equity, and accessibility. “IDEA-led data,” on the contrary, is an evolving term — it is about the usage of data in a way that is grounded in the intentions of inclusion, diversity, equity, and accessibility. This usage of data can be in any shape —collection, analysis, strategy development, etc. “IDEA data” can be seen as a subset of “IDEA-led data.”
I must admit that I deeply appreciate the responses we generate as a group in this process. Each response is unique, and yet, all of them together gives “equity” in data a meaning.
See it this way, ask every household member to clean the messiest room and observe how they do it. I can guarantee they won’t have the same starting point in cleaning that room. Some will start with folding laundry; others might start with picking out all empty bottles, cans, or products in the room. That’s what it is like to learn advancing equity in data. That journey is unique and personal — and it starts with learning about diversity, access, and voice. There is no one checklist of “how-to” here. It starts with asking ourselves — what is our purpose and intention with the data? Does it perpetuate harm or create opportunities of equity and inclusion?
2. Identity is very personal (and fluid at times) information. There is no one right way to capture it, except that it has to be human.
Acknowledge that this is personal and fluid information (fluid because identity information can change). That means it should be a non-negotiable priority to design the questions in a way that centers the people responding to those questions in your data collection exercise.
For example, the questions around gender, race, and sexual orientation are often offered in limited 5-7 checkbox options. Identity is extremely personal information about someone, and 5-7 options may not cover everyone’s personal identity.
There is missing and broken trust in how we have collected and counted data in the past. And, designing those questions, like always, does not generate confidence. After all, it is something for which people continue to be excluded and harmed. Designing data collection questions around identity information thus starts with two fundamentals. One, as the data collection team, being clear about the purpose of the data that is collected. That purpose has to connect with a concrete action. Example: pronouns are asked so they can be used to appropriately acknowledge individuals in written/verbal communication. Two, as designers, being flexible in the way questions are asked so it prioritizes the people who respond. Example: checkbox vs. open-ended identity-based questions. I acknowledge that this second point (being flexible in design) can be tricky at times, and it is something I get to brainstorm with my co-learners.
3. The same data may tell very different stories to each group of people it impacts.
Many years ago, my statistics professor taught us a trick. “Add a quantitative data point and you will generate a reaction.” Years later, I got to use it in my project (and now in this example). Here is an anecdote that I often use and discuss in my workshop:
I am working on a research project that involves evaluating if and how the needs of first-generation immigrants across different generations have changed and shaped the local landscape. That project demands both creativity and patience in data collection (it’s an ongoing[MD1] project). It has everything in the mix – interviews, focus groups, and community surveys.
One of the common questions asked in the interviews and focus groups conducted so far has been, “In 2017, a report published 8 out of 10 employers admit ‘regional accents influence recruitment decisions.’ Do you think accents influence job opportunities as first-generation immigrants?” The response is interesting (on the basis of what is collected as of now). There is a clear difference in the response (verbal and body language) when asked to white, native English speakers vs. non-white, multilingual speakers. Similarly, there is a difference in the response when the respondents were first-generation immigrants across the 70s vs 90s vs 2000s, etc.
Clearly, the same question generates different reactions (and data points).
What I bring back from that example into my workshop discussion is – being mindful about the reports that come out of such data collection instances when there are many different voices in the play. When interpreting and translating that data into insights, it is critical to include:
What is the context here, so we understand qualitative vs. quantitative is better?
- Who collected this data?
- Are there actions on the part of the sponsors in ethical use, distribution, and next steps from this data?
- How was this data collected?
- Who will the insights speak to?
When working with data, there is no specific step where equity needs to be centered; equity should be embedded in the entire process. The point of this conversation is to bring awareness and responsibility to our actions for and from our data.
4. Power is deeply intertwined with language.
Changing the data culture to use data responsibly comes with its roadblocks. Several challenges can surface depending on who is leading those efforts in the organization. Some specific roadblocks could be an inability to get before the right stakeholders, being gaslighted by the right stakeholders, or being shut down by someone who holds more power.
For example, one of the workshop participants once shared how she wanted to update what is collected and reported around the identity of donors, volunteers, and other key groups in her organization. She realized that updates required conversations within and outside her team, so a clear why, what, and how could be collectively established in making those updates. So, she asked for those meetings and space in meeting agendas. But, as an entry-level data coordinator (and a Black woman), she shared how she found resistance to those needed conversations. The updates are still pending and live in an internal tracker.
Each of us is responsible for building a better individual relationship with data. This will look different from role to role. A data analyst will require a different level of understanding in cleaning and analyzing data than a team leader responsible to synthesize a bulky report for the Board. Each will require a different lens on the summarized charts and language of its narratives.
Regardless of how that individual relationship (with data) looks like, the underlying intention here goes back to the first point — reflecting (and acting) on what equity in data means. And, when we make an active effort in understanding “data” and its nuances, we can acknowledge the positional power we have in influencing our data culture.
5. All of us who work in the sector are, to a certain extent, storytellers.
We tell stories using the data we have, for the data we want to collect. We can make those stories inclusive by creating a space of collaboration and feedback with the community whose stories they are while learning to say “I don’t know” at appropriate times.
I particularly love a relevant example I received in the workshop. One of the participants was both an annual-giving fundraiser and the data person in the fundraising team of an independent school. Their mission was to offer learning and development opportunities to kids and youth of low-income families with one or both BIPOC parents. She shared how the impact stories they collected in their data didn’t feel adequate in light of the pandemic challenges, so they changed their process. Instead of looking at their own previously collected data, they opened up the process to include their entire community (families, kids, digital supporters, etc.). Not only did they bring representatives of the community in designing the questions of data collection, but they also supported in summarizing and interpreting the responses to define “impact.”
Remember, the “impact” of the stories is not through the numbers but the people in those stories. In the example above, a fair share of voices were included, right from the way data was collected to how it translated into impact. Let us lean on both the data and the people underlying it.
You and I live in a time when data is constantly and continuously collected about us, through us, claiming it is for us. We need to remember that data in itself is not magic. Just like my Netflix recommendation algorithm was dependent on my (previously) limited engagements on the platform, our data outputs will only be as dynamic as the information we give it. It is not perfect, either. It (data) is merely a recorded value — by a person or a device — in this world.
Our complex and imperfect world.
Learning to form a healthy relationship with data is, therefore, a necessity to building equity. Because that establishes our commitment to count right and our patterns of engagement with data. To be counted in that data should not be a matter of power or privilege for anyone.
It must be fundamental.
[/et_pb_text][/et_pb_column][/et_pb_row]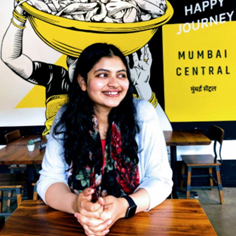
Meenakshi Das
Meenakshi (Meena) Das (she/her/hers) is the founder, data consultant, trainer, and an ethicist with her nonprofit consulting practice, NamasteData. She specializes in designing and teaching equitable research tools and analyzing engagement. Her two workshops referenced in the article are: Advancing Equitable Data Collection and Advancing Equity through Visualizations. Meena appreciates spending her time outside work as a mentor to immigrants. Her recent favorite project is talking about IDEA-led data and research through her LinkedIn-based newsletter data uncollected.
I am a first-generation South Asian immigrant woman. I speak a few different languages and like to keep learning new ones. I never imagined my introduction to the Korean language would be through the amazing K-Dramas I found on Netflix a few years ago. Have you watched any K-Dramas? You should try if you haven’t. Or Turkish dramas. They are good too.
I know this isn’t an essay about TV recommendations (we can do that in our own time).
My point here is that it was not until I watched these shows that I realized I must engage with my Netflix account differently to be able to appreciate content beyond what I (and Netflix’s recommendation algorithm) already knew I liked. And that was one of the life lessons that led me to create my two data equity workshops.
Let me back up a little to expand on that Netflix example: that platform takes pride in the level of personalization and recommendations it can offer its viewers. Until a few years ago, my Netflix “top picks for you” was a list of stories I was comfortable with — Hallmark classics and very specific thrillers. It was not until I deliberately searched and found my first K-Drama that it started to appear on my recommendation list.
Here is the danger of extreme personalization — we risk limiting ourselves to what we know, what we understand, and what makes us comfortable. After “confusing” Netflix’s recommendation algorithm several times, I have learned about horses, street-food business women in South America, the story behind the thickness of Thai noodles, and, of course, more Korean and Turkish dramas.
My workshops to advance equity in data are built with this intention — to build collective knowledge around data collection and visualization in a way that allows us to appropriately challenge those places where data can lead to exclusion and alienation.
I learned five lessons by offering these workshops to individuals from different roles, sectors, and data comfort that I want to share with you. I call those individuals my co-learners.
1. What constitutes “equity” in data results from evolving learning around diversity, access, and voice.
I start the workshop with a basic question — “what does IDEA-led data mean to you?” (IDEA refers to Inclusion, Diversity, Equity, and Accessibility)
This question is primarily for two reasons:
- I want my co-learners to actively reflect on their lived and learned experiences, so they realize how their (and their community’s collective) privileges and challenges are counted or eliminated from the data, and
- I want each of us to remember and be able to distinguish between “IDEA data” and “IDEA-led data.”“IDEA data” is a clear term — it is the data about inclusion, diversity, equity, and accessibility. “IDEA-led data,” on the contrary, is an evolving term — it is about the usage of data in a way that is grounded in the intentions of inclusion, diversity, equity, and accessibility. This usage of data can be in any shape —collection, analysis, strategy development, etc. “IDEA data” can be seen as a subset of “IDEA-led data.”
I must admit that I deeply appreciate the responses we generate as a group in this process. Each response is unique, and yet, all of them together gives “equity” in data a meaning.
See it this way, ask every household member to clean the messiest room and observe how they do it. I can guarantee they won’t have the same starting point in cleaning that room. Some will start with folding laundry; others might start with picking out all empty bottles, cans, or products in the room. That’s what it is like to learn advancing equity in data. That journey is unique and personal — and it starts with learning about diversity, access, and voice. There is no one checklist of “how-to” here. It starts with asking ourselves — what is our purpose and intention with the data? Does it perpetuate harm or create opportunities of equity and inclusion?
2. Identity is very personal (and fluid at times) information. There is no one right way to capture it, except that it has to be human.
Acknowledge that this is personal and fluid information (fluid because identity information can change). That means it should be a non-negotiable priority to design the questions in a way that centers the people responding to those questions in your data collection exercise.
For example, the questions around gender, race, and sexual orientation are often offered in limited 5-7 checkbox options. Identity is extremely personal information about someone, and 5-7 options may not cover everyone’s personal identity.
There is missing and broken trust in how we have collected and counted data in the past. And, designing those questions, like always, does not generate confidence. After all, it is something for which people continue to be excluded and harmed. Designing data collection questions around identity information thus starts with two fundamentals. One, as the data collection team, being clear about the purpose of the data that is collected. That purpose has to connect with a concrete action. Example: pronouns are asked so they can be used to appropriately acknowledge individuals in written/verbal communication. Two, as designers, being flexible in the way questions are asked so it prioritizes the people who respond. Example: checkbox vs. open-ended identity-based questions. I acknowledge that this second point (being flexible in design) can be tricky at times, and it is something I get to brainstorm with my co-learners.
3. The same data may tell very different stories to each group of people it impacts.
Many years ago, my statistics professor taught us a trick. “Add a quantitative data point and you will generate a reaction.” Years later, I got to use it in my project (and now in this example). Here is an anecdote that I often use and discuss in my workshop:
I am working on a research project that involves evaluating if and how the needs of first-generation immigrants across different generations have changed and shaped the local landscape. That project demands both creativity and patience in data collection (it’s an ongoing[MD1] project). It has everything in the mix – interviews, focus groups, and community surveys.
One of the common questions asked in the interviews and focus groups conducted so far has been, “In 2017, a report published 8 out of 10 employers admit ‘regional accents influence recruitment decisions.’ Do you think accents influence job opportunities as first-generation immigrants?” The response is interesting (on the basis of what is collected as of now). There is a clear difference in the response (verbal and body language) when asked to white, native English speakers vs. non-white, multilingual speakers. Similarly, there is a difference in the response when the respondents were first-generation immigrants across the 70s vs 90s vs 2000s, etc.
Clearly, the same question generates different reactions (and data points).
What I bring back from that example into my workshop discussion is – being mindful about the reports that come out of such data collection instances when there are many different voices in the play. When interpreting and translating that data into insights, it is critical to include:
What is the context here, so we understand qualitative vs. quantitative is better?
- Who collected this data?
- Are there actions on the part of the sponsors in ethical use, distribution, and next steps from this data?
- How was this data collected?
- Who will the insights speak to?
When working with data, there is no specific step where equity needs to be centered; equity should be embedded in the entire process. The point of this conversation is to bring awareness and responsibility to our actions for and from our data.
4. Power is deeply intertwined with language.
Changing the data culture to use data responsibly comes with its roadblocks. Several challenges can surface depending on who is leading those efforts in the organization. Some specific roadblocks could be an inability to get before the right stakeholders, being gaslighted by the right stakeholders, or being shut down by someone who holds more power.
For example, one of the workshop participants once shared how she wanted to update what is collected and reported around the identity of donors, volunteers, and other key groups in her organization. She realized that updates required conversations within and outside her team, so a clear why, what, and how could be collectively established in making those updates. So, she asked for those meetings and space in meeting agendas. But, as an entry-level data coordinator (and a Black woman), she shared how she found resistance to those needed conversations. The updates are still pending and live in an internal tracker.
Each of us is responsible for building a better individual relationship with data. This will look different from role to role. A data analyst will require a different level of understanding in cleaning and analyzing data than a team leader responsible to synthesize a bulky report for the Board. Each will require a different lens on the summarized charts and language of its narratives.
Regardless of how that individual relationship (with data) looks like, the underlying intention here goes back to the first point — reflecting (and acting) on what equity in data means. And, when we make an active effort in understanding “data” and its nuances, we can acknowledge the positional power we have in influencing our data culture.
5. All of us who work in the sector are, to a certain extent, storytellers.
We tell stories using the data we have, for the data we want to collect. We can make those stories inclusive by creating a space of collaboration and feedback with the community whose stories they are while learning to say “I don’t know” at appropriate times.
I particularly love a relevant example I received in the workshop. One of the participants was both an annual-giving fundraiser and the data person in the fundraising team of an independent school. Their mission was to offer learning and development opportunities to kids and youth of low-income families with one or both BIPOC parents. She shared how the impact stories they collected in their data didn’t feel adequate in light of the pandemic challenges, so they changed their process. Instead of looking at their own previously collected data, they opened up the process to include their entire community (families, kids, digital supporters, etc.). Not only did they bring representatives of the community in designing the questions of data collection, but they also supported in summarizing and interpreting the responses to define “impact.”
Remember, the “impact” of the stories is not through the numbers but the people in those stories. In the example above, a fair share of voices were included, right from the way data was collected to how it translated into impact. Let us lean on both the data and the people underlying it.
You and I live in a time when data is constantly and continuously collected about us, through us, claiming it is for us. We need to remember that data in itself is not magic. Just like my Netflix recommendation algorithm was dependent on my (previously) limited engagements on the platform, our data outputs will only be as dynamic as the information we give it. It is not perfect, either. It (data) is merely a recorded value — by a person or a device — in this world.
Our complex and imperfect world.
Learning to form a healthy relationship with data is, therefore, a necessity to building equity. Because that establishes our commitment to count right and our patterns of engagement with data. To be counted in that data should not be a matter of power or privilege for anyone.
It must be fundamental.
[/et_pb_text][/et_pb_column][/et_pb_row]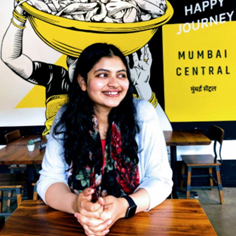
Meenakshi Das
Meenakshi (Meena) Das (she/her/hers) is the founder, data consultant, trainer, and an ethicist with her nonprofit consulting practice, NamasteData. She specializes in designing and teaching equitable research tools and analyzing engagement. Her two workshops referenced in the article are: Advancing Equitable Data Collection and Advancing Equity through Visualizations. Meena appreciates spending her time outside work as a mentor to immigrants. Her recent favorite project is talking about IDEA-led data and research through her LinkedIn-based newsletter data uncollected.
Discover more from CCF
Subscribe to get the latest posts sent to your email.
Dear Meena,
This is a very well-written piece!! I especially liked how you start with a very relatable example and brought the article full circle by bringing it back.
Great job once again! I already shared it at my workplace and I look forward to sharing this further to my network.
Thank you so much for this article. It helped me to look at a piece of data we are hoping to collect more critically.
Thank you, Xin-Yi. Appreciate it so much! <3